Our brains are wired to offload effort, and tools like AI systems are taking this to a whole new level. This natural ability to share our thinking with tools, people, and technology is the essence of distributed cognition — opening up thrilling possibilities for collaboration and innovation.
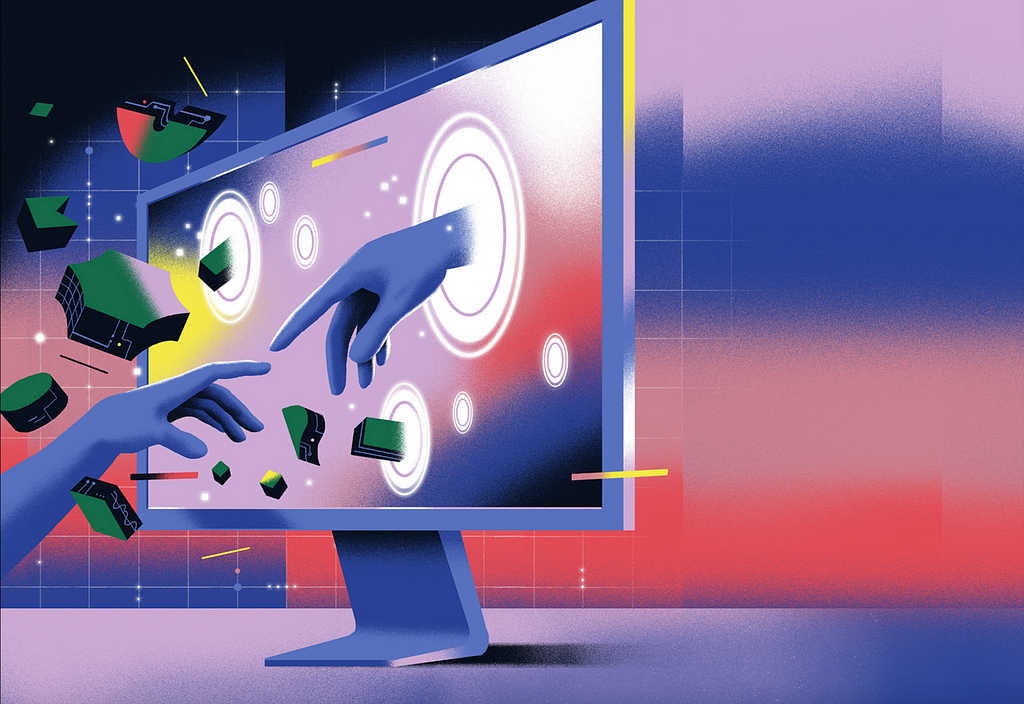
Designing for externalized thought
Our brains are masterful delegators. We offload cognitive work onto everything from sticky notes to sophisticated software. This natural tendency to distribute our thinking across people, tools, and the environment is the essence of distributed cognition. It challenges the traditional view of the mind as an isolated entity, recognizing that our cognitive processes extend beyond our individual skulls.
Imagine you’re driving to a new restaurant with a friend. You’re behind the wheel, your friend navigates using their phone. Who’s really doing the “thinking” here? It’s a collaborative effort, a distributed cognitive system encompassing both individuals and technology. Just as a pilot relies on instruments and air traffic control, we all leverage external resources to think, learn, and solve problems.
This principle of distributed cognition is particularly relevant in today’s world, where we increasingly interact with AI systems that become integral parts of our cognitive processes. We need to design for the system of thinking, recognizing the dynamic interplay between individuals, AI agents, and the surrounding context. This isn’t just about reducing cognitive burden; it’s about empowering users to think and work more effectively. By designing systems that seamlessly integrate AI into human cognitive processes, we can create tools that amplify human potential and redefine what it means to collaborate with technology.
The origins of distributed cognition
How do people on a navy ship navigate the ocean? It’s not just the captain at the helm or a navigator with a map — it’s an intricate dance of people, tools, and time, working together in perfect sync. This is the essence of distributed cognition, a term introduced by Edwin Hutchins in his groundbreaking work Cognition in the Wild. Hutchins didn’t just theorize; he observed real-world navigation processes, uncovering how cognition isn’t confined to individual minds but is spread across systems of humans and tools.
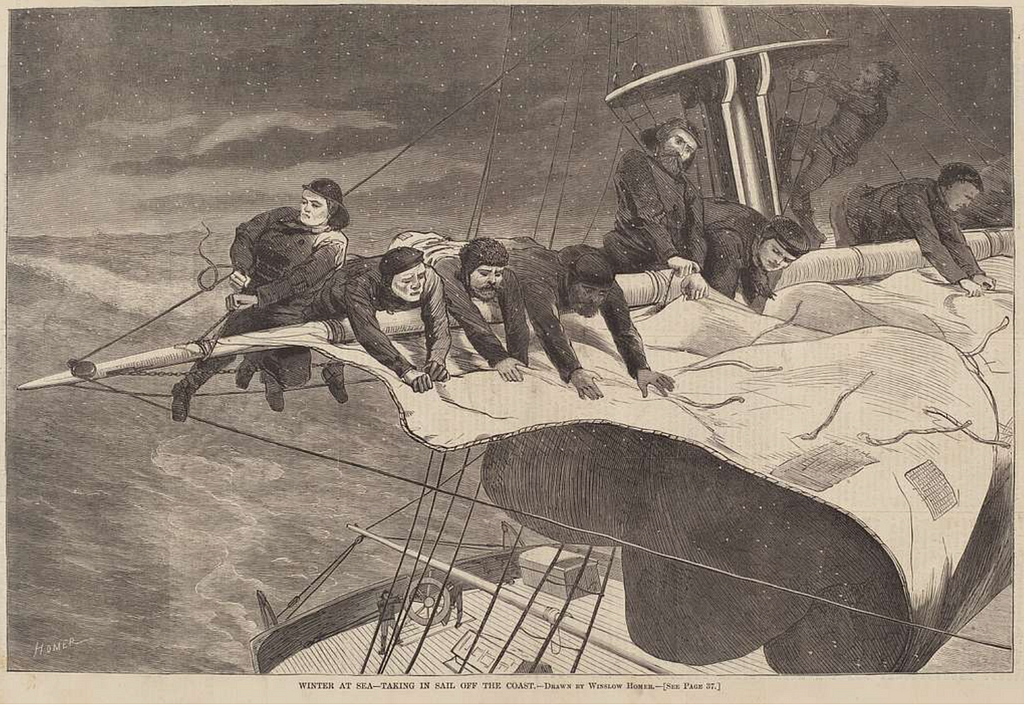
Hutchins’ studies revealed that naval officers’ cognitive activities relied heavily on two things: social interactions and precise use of navigational tools. From these observations, he argued that real-world cognition isn’t just about internal thought processes, as traditional theories like the symbolic paradigm suggest.
Instead, it’s a systemic activity distributed across three key dimensions:
- Humans and their environment — Cognition flows between people and the physical tools they use, like compasses, maps, or modern GPS systems.
- Multiple individuals — Thinking often emerges from collaboration, with ideas bouncing between team members in dynamic interactions.
- Time — Cognition builds on cultural artifacts and knowledge passed down from prior generations, creating a temporal thread of problem-solving.
Hutchins proposed that to truly understand thinking, we must shift our focus. Rather than isolating the mind as the primary unit of analysis, we should examine the larger sociotechnical systems in which cognition occurs. These hybrid systems blend people, tools, and cultural artifacts, creating a web of interactions that extend far beyond any single individual.
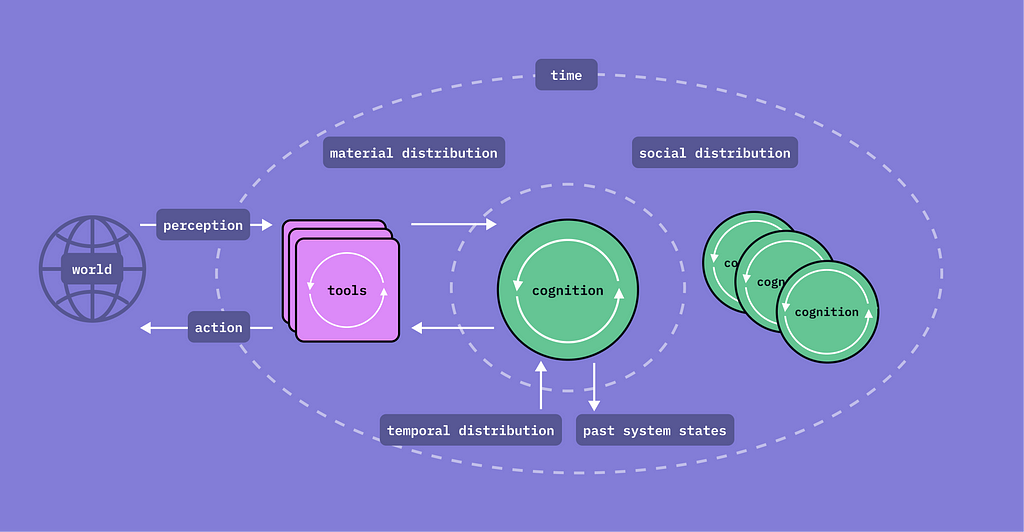
That was the ’90s. Today, modern information technologies, especially AI, are revolutionizing the way we think and work by transforming how cognitive processes are distributed. Computers and AI systems take on repetitive and tedious tasks — like calculations, data storage, and searching — freeing humans to focus on creativity and problem-solving. But they don’t just lighten our mental load; they expand and enhance our cognitive abilities, enabling us to tackle problems and ideas in entirely new ways.
Humans and computers together form powerful hybrid teams. As famously (but wrongly) credited to Einstein: “Computers are fast, accurate, and stupid; humans are slow, brilliant, and together, unstoppable.”
Today, this partnership has opened up three key pathways for cognition:
- Human-only cognition: When people rely solely on their minds without external technology.
- Digitally supported cognition: Where humans and computers collaborate, with digital tools amplifying human intelligence.
- Autonomous AI systems: Where cognition is fully externalized into machines, allowing AI to operate independently of human input.
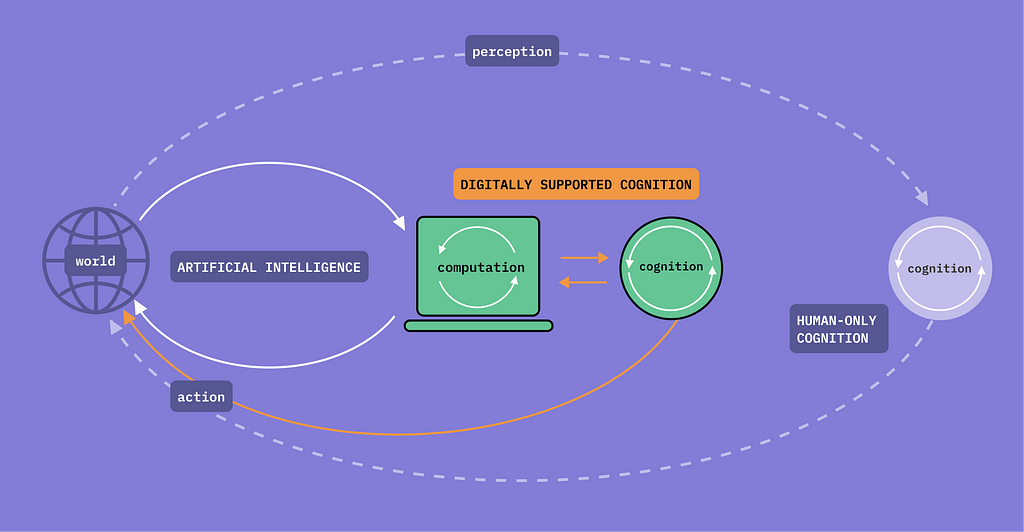
Cognitive offloading — the thinking partnership
Building on the idea of distributed cognition, where thinking emerges through the interplay of minds, tools, and environments, one key principle stands out: the concept of cognitive offloading. This refers to the way humans offload mental tasks onto the environment, using artifacts, technologies, and even other people to reduce the burden on their cognitive systems and enhance their ability to think, reason, and solve problems.
Sense-making — whether it’s tackling a complex problem, brainstorming ideas, or navigating everyday challenges — doesn’t happen in isolation. Instead, it unfolds in collaboration with the world. By perceiving, exchanging, and manipulating information in hybrid systems of human-tool or human-technology interactions, we extend the boundaries of cognition far beyond the brain. Think of using a calculator to solve a math problem, or a collaborative app to plan a project. These are examples of offloading, where tools become partners in the cognitive process.
Designing for cognitive offloading is crucial, especially in AI applications where users interact to form digitally supported cognition. The goal is to create environments that not only facilitate offloading, but also empower users to seamlessly and effectively extend their thinking.
Principles of designing for cognitive offloading
Let’s take a closer look at some standout AI applications that excel at managing cognitive offloading and explore the key principles behind their effectiveness.
1. Understanding user pain points
Cognitive effort can feel like a form of pain. Mental effort is intrinsically costly, as it demands a significant amount of cognitive resources simply due to the way our brains function. This is rooted in how the brain manages energy — mental effort consumes glucose, the brain’s energy source, which can lead to fatigue and feelings of discomfort. This discomfort is often experienced as mental resistance or even avoidance, which explains why people are prone to procrastinate on mentally taxing tasks. Temporal Motivation Theory also supports it, stating that tasks perceived as difficult or unenjoyable, especially without immediate rewards, are likely to be postponed.
Users are naturally drawn to tools that minimize this sense of effort.
To design effectively, start by identifying pain points: observe user behavior, conduct interviews, and uncover the cognitive tasks that slow them down. Then, design features that seamlessly offload these burdens.
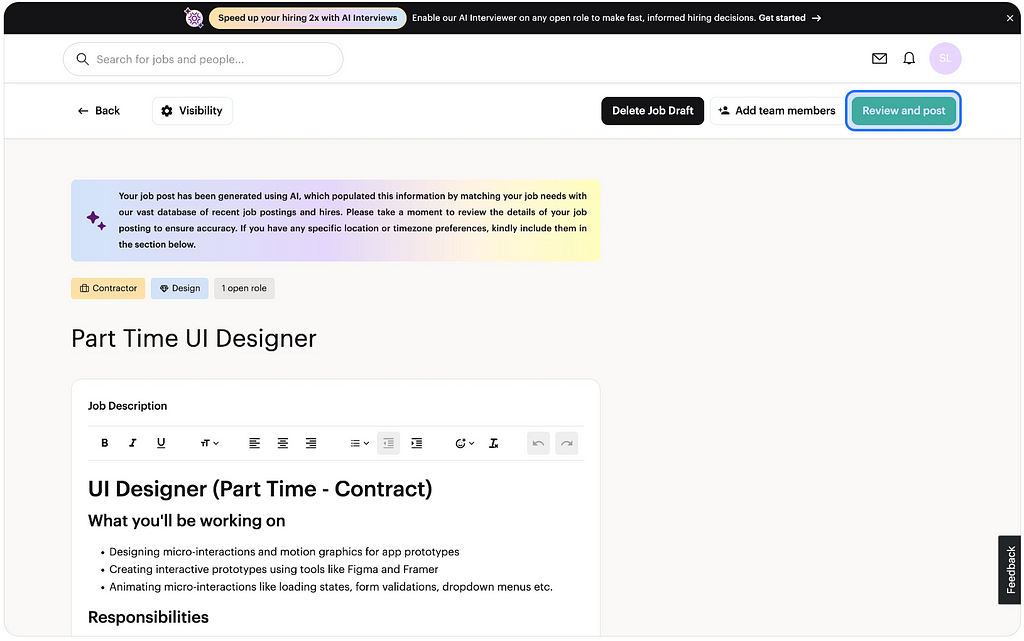
For example, Braintrust excels in helping users create job descriptions. By leveraging natural language input, the platform transforms a simple brief into a polished posting, eliminating the tedious mental effort of crafting one from scratch. The tool doesn’t just save time; it also reduces decision fatigue by guiding users through the process intuitively, ensuring that key details are captured without requiring extensive knowledge or expertise in job writing.
This approach empowers users by providing a framework they can build upon, blending automation with customization. Users can focus on refining and personalizing the output rather than starting from a blank page, making the process more efficient and less stressful.
Some resources:
- “How to make your AI project a success” — IxDF masterclass by Greg Nudelman. Among other things, Greg shares his approach to design the initial UX and importance of real-world constraints and value-based metrics.
- “How to design experiences for AI” — another IxDF masterclass by Greg Nudelman. Key takeaway from me in this one is how to adapt design process for AI.
- UX for AI — a website curated by Greg Nudelman, featuring a collection of best practices, insightful talks, and opinion pieces on AI design. I recommend joining his mailing list to stay updated on new articles and resources.
- “Doing UX research in the AI space” — an article from GitLab handbook with practical tips on how to conduct UX research in the AI space, including research guidelines.
2. Promoting collaborative problem-solving
Users naturally benefit from tools that enable collaboration, especially when they help to offload cognitive tasks that might otherwise slow them down. Collaborative systems bridge the gap between human creativity and machine efficiency, lightening the mental load while enhancing productivity.
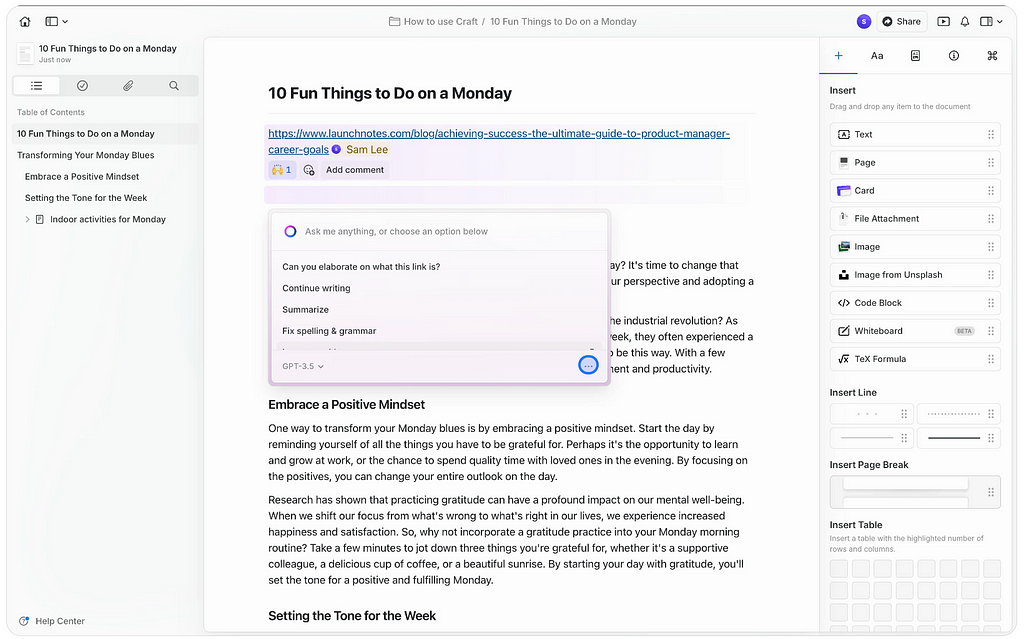
For example, Craft App uses AI functionality to help users overcome writer’s block. When a user pauses or struggles to move forward, the app offers intelligent suggestions or continues the text seamlessly. This reduces the cognitive effort required to generate ideas from scratch, allowing users to focus on shaping and refining their thoughts. The collaboration feels intuitive, with the AI stepping in as a partner to share the cognitive load rather than leaving the user to face the challenge alone. By doing so, the tool creates an environment where creative flow is easier to sustain, enabling users to think and work more freely.
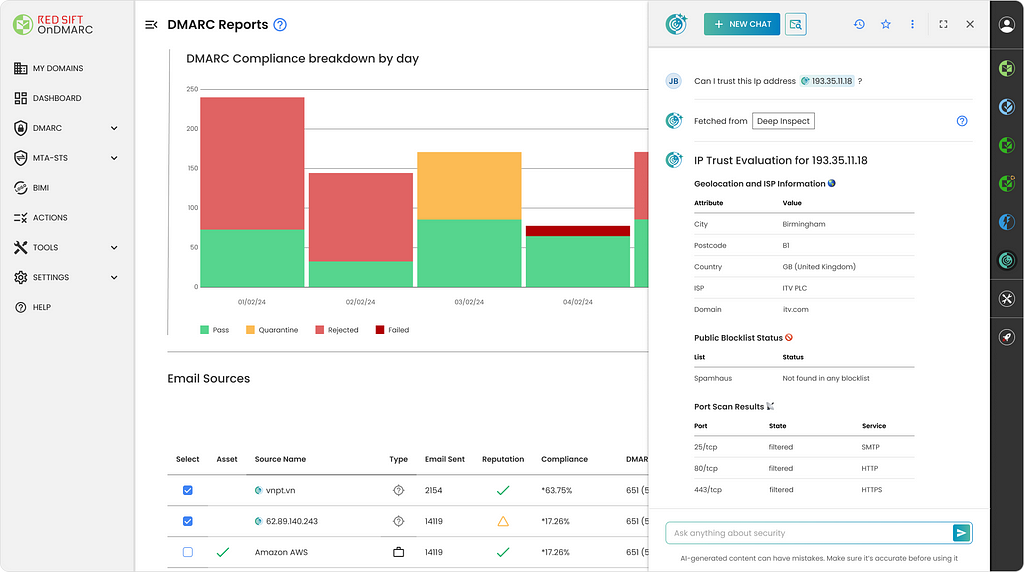
Red Sift Radar provides another strong example of collaboration that facilitates cognitive offloading. In this workflow, an AI copilot indicator appears next to a domain’s IP address, signaling interactivity. Evaluating the trustworthiness of a domain is a common task users perform on this screen, and the AI copilot anticipates this need, seamlessly providing a shortcut. With a simple click, users can ask questions about the selected domain and, among other things, assess its trustworthiness — an otherwise time-consuming task if done manually. This collaborative approach allows the AI to handle the heavy lifting, streamlining the process and empowering users to make informed decisions quickly and efficiently.
Some resources:
- Microsoft Research AI Frontiers are sharing some research papers on Human-Computer Interaction in Publications
- My top recommendation from them is “Guidelines for Human-AI Interaction” (2019) — a must-read.
3. Using existing mental models and patterns
According to Jakob’s Law, “Users spend most of their time on other sites. This means they prefer your site to work the same way as all the other sites they already know.” This principle extends beyond websites to any interface users interact with. Leveraging mental models and established patterns is a golden rule for reducing cognitive load, as it allows users to apply their prior experiences seamlessly.
“As you onboard users to a new AI-driven product or feature, guide them with familiar touchpoints.
With AI-driven products, there can be a temptation to communicate the “newness” or “magic” of the system’s predictions through its UI metaphors.
However, unfamiliar UI touchpoints can make it harder for users to learn to use your system, potentially leading to degraded understanding of, or trust in, your product, no matter the quality of your AI output.
Instead, anchor new users with familiar UI patterns and features. This will make it easier for them to focus on the key task at hand, which is building comfort with, and calibrating their trust in, your system’s recommendations”.
People + AI Guidebook by Google
For example, Relume uses purple accents and sparkle emojis to highlight AI-powered features, which have already become an established pattern for communicating AI-supported functionalities, especially in products that are not AI-first. This approach facilitates cognitive offloading by reducing the mental effort required for users to identify and engage with AI functionalities. Instead of scanning the interface or reading detailed instructions, users can rely on these visual cues to quickly locate AI tools.
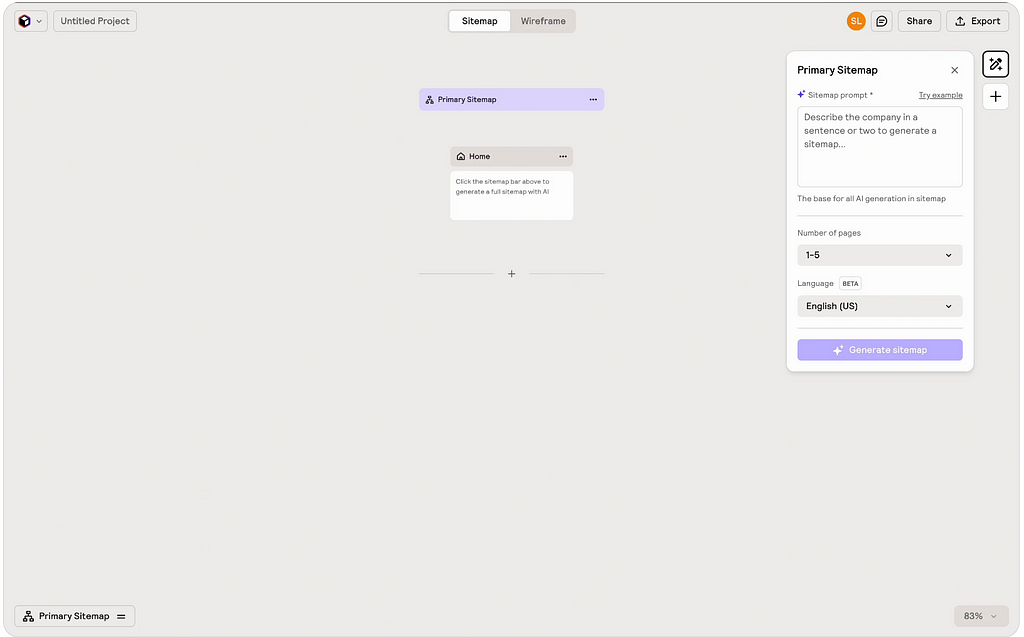
Some resources:
- “UI design patterns for successful software” — before exploring AI-specific patterns, it’s a good idea to refresh your understanding of standard ones, and this IxDF course is an excellent way to do so.
- While the design rules for AI are still being written, you can spot emerging trends on the “Shape of AI” website — a treasure trove of examples curated by Emily Campbell
- Vitaly Friedman also shared his observations in IxDF masterclass “How to elevate the user experience of AI with design patterns”
- The People + AI Research team at Google developed a list of patterns as part of their Guidebook — a comprehensive resource featuring methods, best practices, and examples for designing with AI.
4. Leveraging progressive disclosure
Progressive disclosure is a classic principle in human-computer interaction that simplifies complex interactions by introducing content and functionality in incremental steps. Instead of overwhelming users with all options at once, this approach reveals information as needed, based on the user’s progress through the system. This method is particularly effective in AI applications, where managing complexity is crucial.
For example, Airtable’s AI-assisted app creation flow uses a wizard-like interface, guiding users through bite-sized, context-aware steps. By tailoring the experience to the user’s specific goals, Airtable eliminates the cognitive load of filling out endless forms, making the process feel intuitive and manageable.
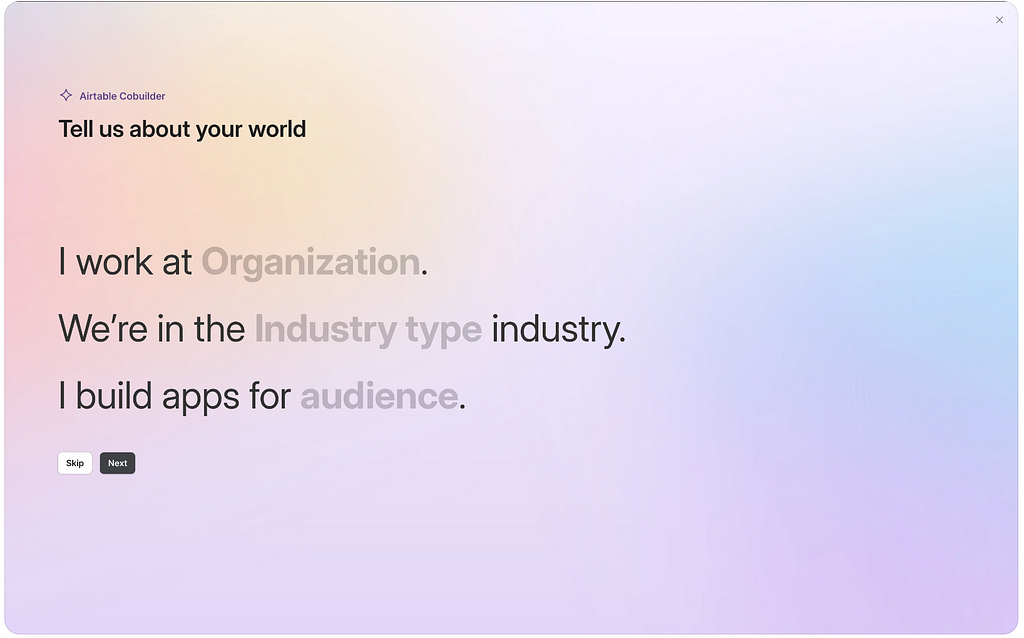
Another great example is Jasper, which uses tiles to present snippets of functionality. Rather than overloading users with the technical details of how text is generated, Jasper focuses on identifying the end goal of the collaboration between human and AI. This allows users to concentrate on outcomes, not processes, creating a seamless and engaging experience that reduces cognitive effort.
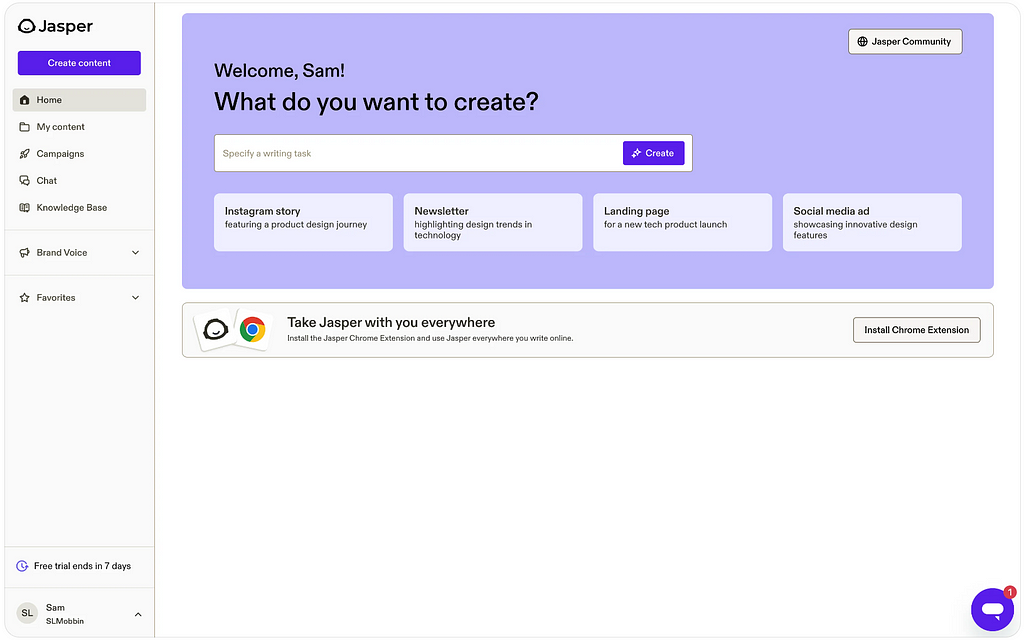
Some resources:
- “Progressive disclosure” — an article providing a detailed overview of the technique by Jakob Nielsen.
- “Progressive disclosure” — an article by Frank Spiller from The Glossary of Human Computer Interaction book.
- “Progressive disclosure: when, why, and how do users want algorithmic transparency information?” — a research paper exploring how to balance explainability with cognitive overload.
- “Progressive disclosure options for improving choice overload on home screens” — a research paper that connects progressive disclosure with the concepts of choice overload and legibility, offering some practical insights.
5. Ensuring graceful failure
AI functionality is still new to many users, and errors are inevitable. The key is to ensure that mistakes don’t disrupt the user flow entirely. Instead, the system should support users in recovering smoothly and continuing toward their goal. This is especially critical for AI systems, where trust can be fragile — just one glaring error can be enough to break a user’s confidence in the tool.
Graceful failure means designing systems that handle errors transparently and helpfully. For example, if an AI misinterprets a query or generates a flawed result, it should provide users with an easy way to revise, retry, or understand what went wrong. By offering clear explanations, alternative suggestions, or fallback options, AI systems can maintain user trust and engagement, even when things go awry.
A great example of ensuring graceful failure is Google Search’s AI-powered feature, such as ‘These are results for [corrected term].’ When a user misspells a query or submits something unclear, the system provides corrections or alternative results instead of returning irrelevant or empty pages. This approach gently guides users to relevant results, helping them recover from input errors without disrupting their flow.
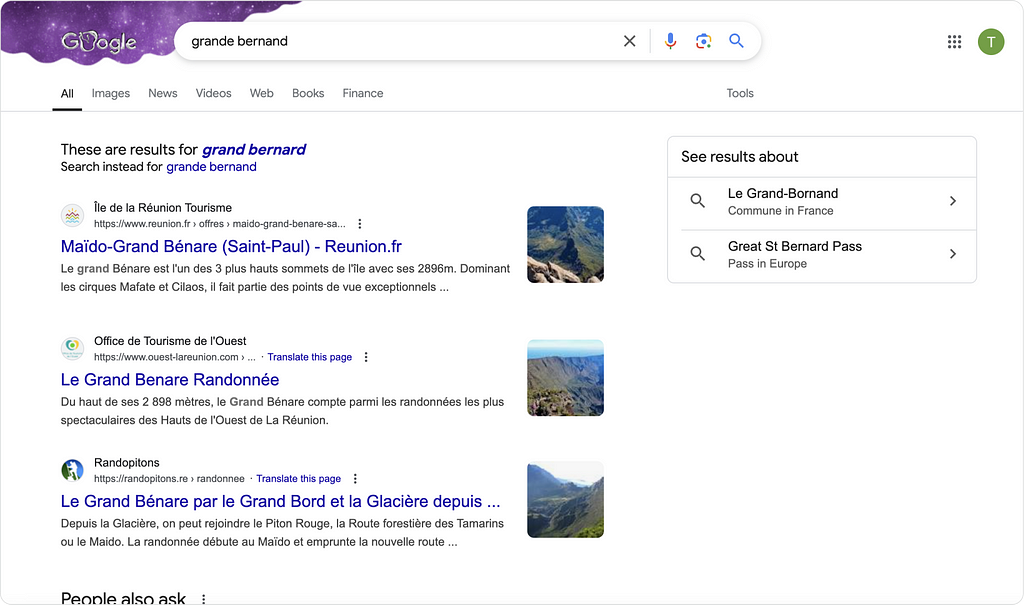
Some resources:
- “The importance of graceful degradation in accessible interface design” — an article by Eleanor Hecks on Smashing Magazine
- “The art of failing gracefully” — an article by Solomon Hawk that explores both engineering and UX perspectives.
- An article “Errors + Graceful Failure” from People + AI Guidebook
6. Contextual guidance
The success of human-AI collaboration hinges on shared mental models between the human and the AI as a hybrid team. For this collaboration to work effectively, users need a clear understanding of the AI system’s capabilities and how to interact with it to achieve their goals. However, expecting users to develop this mental model by reading through a lengthy knowledge base is unrealistic.
A more effective approach is to provide contextual guidance — offering help and explanations exactly when and where users need them. By integrating tooltips, inline prompts, or interactive tutorials, systems enable users to learn naturally as they engage with the interface.
Contextual guidance supports cognitive offloading by reducing the mental effort needed to figure out how the system works. Instead of requiring users to remember multiple potential interactions or features, the system provides timely assistance, guiding them step by step and allowing them to concentrate on achieving their goals. This approach keeps users focused on their tasks while gradually building their understanding of the system’s capabilities.
Providing suggestions is one of the most common ways to help users build a mental model of how an AI system works. By offering relevant prompts, users can quickly grasp the system’s capabilities and the types of interactions it supports. For example, in June, after connecting a data source and viewing their metrics, users can ask the system for insights. To guide this process, June AI provides starting prompts, giving users a clear idea of the types of questions they can ask. These suggestions make it easier for users to explore the system’s functionality, fostering a better understanding of how to interact with it effectively.
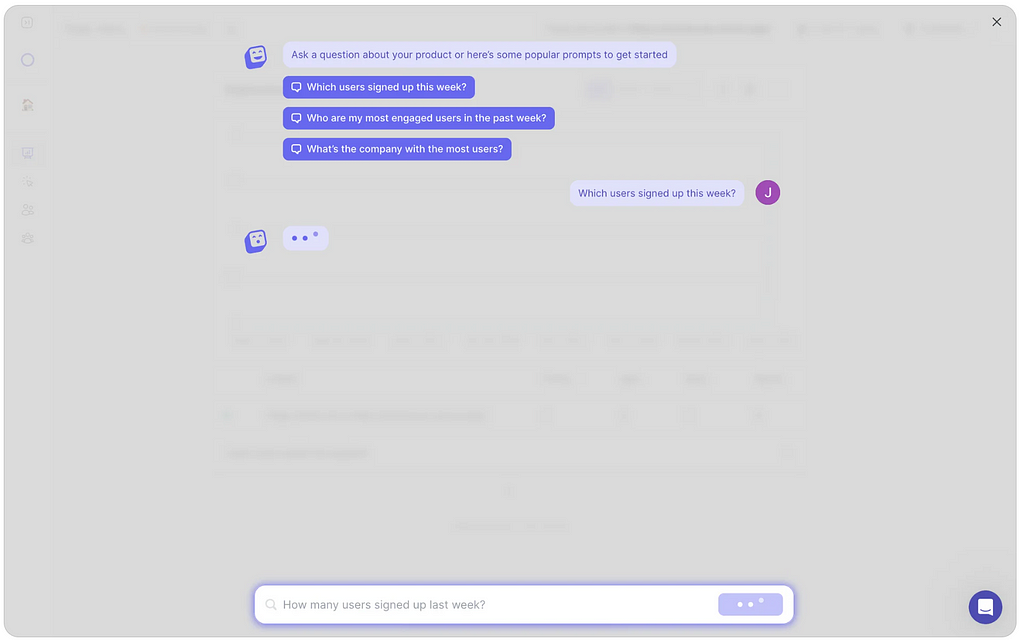
Some resources:
- “Mental models” — an article from People + AI Guidebook
- “Understanding user mental models around AI” — an article by Joana Cerejo
- “Building AI systems with a holistic mental model” — an article by Dr. Janna Lipenkova
- “Generative AI is reshaping our mental models of how products work. Product teams must adjust” — an article by People + AI Research @ Google
Have some more ideas or examples? Share them in the comments!
Final thoughts
Designing systems that enable users to offload cognitive tasks opens up a world of exciting possibilities. By reducing the burden of tedious work, these systems allow individuals to focus on what truly matters — addressing meaningful challenges. However, it is essential to carefully consider the risks involved, particularly the potential for overreliance on such tools.
Take calculators in schools, for example. When they were first introduced, some teachers went on strike, worried that students would lose essential math skills by depending too much on technology. Decades later, the jury’s still out — did calculators help or hurt the way we learn math? What’s clear is that they changed education forever.
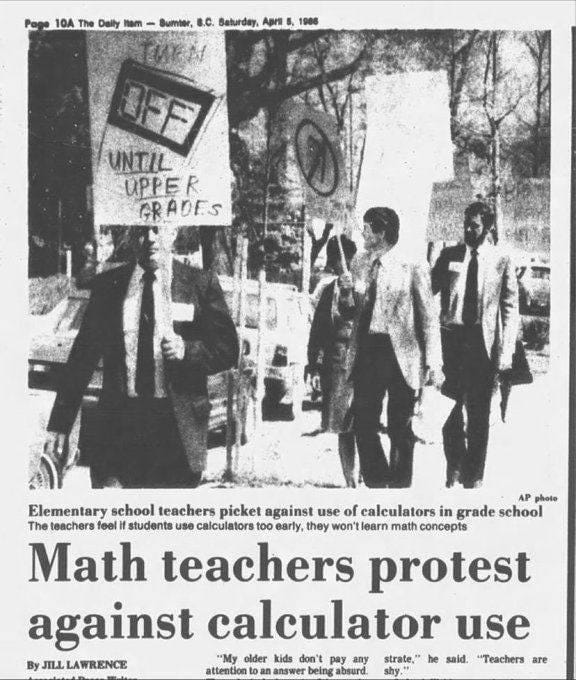
This story reminds us that while tools designed for offloading can make life easier, they must be used thoughtfully. The goal isn’t to let machines think for us, but to work with them to amplify our abilities. Finding that balance ensures we get the best of both worlds — powerful tools that help us grow, without losing the skills that make us uniquely human.
AI and cognitive offloading: sharing the thinking process with machines was originally published in UX Collective on Medium, where people are continuing the conversation by highlighting and responding to this story.
Leave a Reply