Synthetic insights are not research. They are the fastest path to destroy the margins on your product.
There is an invisible reader of every article, whose gaze counts for far more than 1 view, 1 read, or 1 “like.” That reader is, of course, the algorithm.
Between search engines’ page-ranking algorithms and the algorithmic feeds of closed platforms, its silent influence warps the way we write: shorter articles, more frequent publication, more links, and more images. We write for humans, but if we want humans to see what we’ve written, we must write for machines first.
In a way, the paradigm of UX is the opposite. We create experiences for users (it’s in the name) and technology is leveraged in service of that goal. If the product doesn’t do the job, the user will fire it and pick another.
So imagine my frustration as design, with no omnipotent stakeholder to appease, knuckles under the algorithm voluntarily. Design leaders are increasingly advocating for substituting parts of the design process with AI, on the basis that it’s “better than nothing” or “more efficient” (conveniently forgetting who is responsible for design having that “nothing” in the first place).
The whole point of design is solving the problems that linear, mechanical thinking can’t. If we normalize turning to LLMs for our understanding of user needs, we give up our claim on being “champions for the user” and set off a race to the bottom that will see the design process, just like the publishing process, taken over by AI-generated sludge.
Appeals to quality, accuracy, and so forth haven’t worked for writers, and won’t work for designers. Only one argument will: Algorithm-driven design will lose companies a lot of money.
Software-as-a-business
“Design is only as human-centered as the business model allows.” –Erika Hall
Just like everything else about AI tooling, this is not a new conversation but rather a continuation of a very old one. Namely: designers cost money, and so they better make us some money.
So let’s talk about the money. Businesses make money when they sell (or in the case of SaaS, rent out) something that they produce. But outputs aren’t born equal. The important distinction for our purposes is between products and commodities. Commodities, unlike products, are fungible — every sack of grain or bag of concrete is essentially the same, while even something as standardized as a web browser or text editor will have people up in arms if you so much as suggest that they are interchangeable.
Tech made its trillions in the product game, convincing customers that the output they were selling was innovative, unique, never seen before. They could charge an enormous markup for products that couldn’t simply be compared feature-by-feature to competitors, and invest the profits into hiring employees who were innovators and could come up with more unique, never-seen-before ideas to sell.
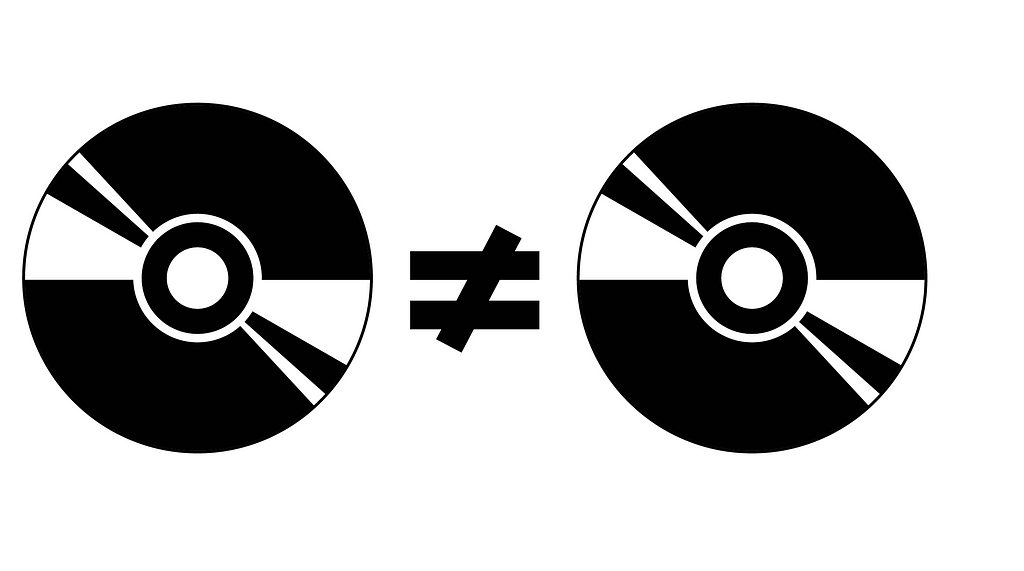
In the commodity game, on the other hand, markup is impossible. When your 2×4 wooden board is identical to your competitor’s 2×4 wooden board, you pretty much have to charge the same price, and the only way to increase your profit is by decreasing the cost per unit. Driving efficiency.
What do you think will happen if all the tech companies outsource their idea generation to the same place? Trying to double down on efficiency by substituting unique human innovators with interchangeable insights from a commoditized robot will turn their software into commodities as well.
Design in the age of algorithmic reproduction
“What is really jeopardized by reproduction is the authority of the object.” — Walter Benjamin
There are two objections that (much like GPT outputs) are so common they are themselves practically commodities, that inevitably crop up in defense of AI “design.” AI skeptics will say: We will only use the LLM as a starting point, or to bounce off ideas, and verify its work. And AI boosters will say: Our model won’t have this problem, it will be uniquely high-quality because we will train it ourselves.
These positions may seem on opposite ends of the spectrum, but they can be brushed away with the same answer: product orgs don’t work that way.
One of my favorite questions to ask in design critique is “what do users do today?” It is an instructive question to ask here.
Do product teams maintain careful decision provenance so that they can remember where their facts came from and what decisions relied on them, or do they suffer from source amnesia where assumptions made years ago become entrenched as facts?
Do product teams do research to disprove their assumptions, or solely to validate them? Do product teams treat research as a loop, or a phase that, once done, need not be revisited again?
And what kind of people are looking to AI for help: the ones with the robust research process, or the ones most interested in skipping due diligence? The ones who are willing to make a colossal investment into their research practice, or the ones that want to cut budgets and corners?
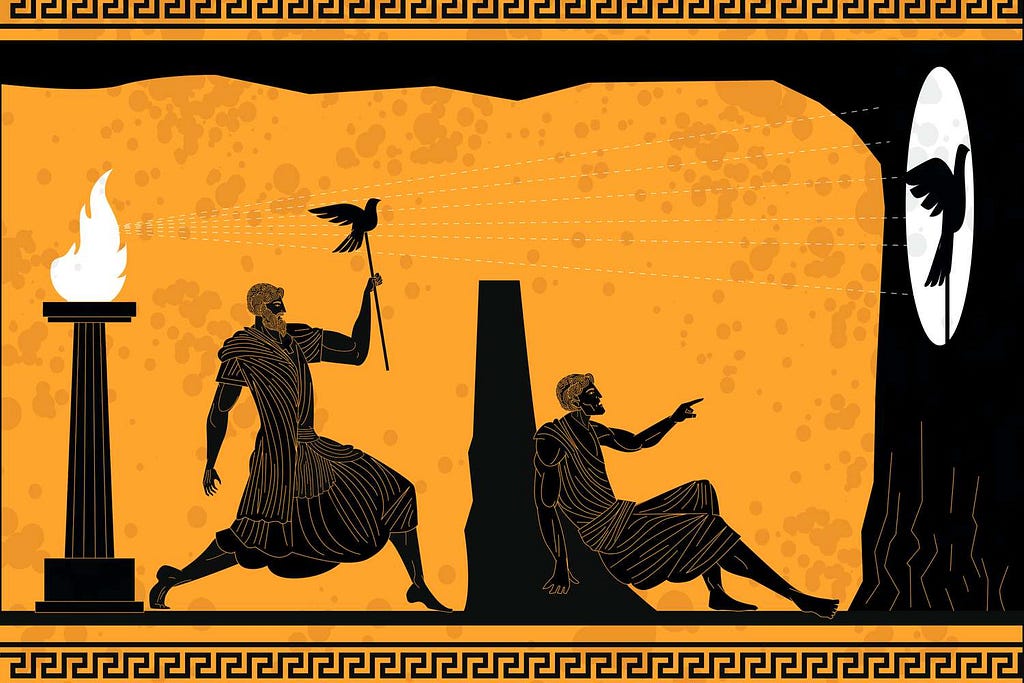
The purpose of a system is what it does
“The repercussions of bad research are more severe than you might imagine.” — Martiina Gilchrist
Ultimately, the decision about whether or not to “streamline” research comes back to the same notorious question asked about design: what is the ROI? What’s so valuable about research that we have to spend time and money on it?
The answer is: the voice of the customer. But I’m using that term a little bit more literally than most.
What’s normally referred to as “voice of the customer” is “phrasing statements that sound like our customer would say.” The problem with that view is, as any user researcher knows all too well, “would” is not reliable. We don’t ask “would you..?” — we ask “how have you…?” The only voice of the customer that really matters is what actual customers — with eyeballs and index fingers and wallets — have said. Verbatim.
Execs often recoil when they hear this, claiming “we need to stay broad to maximize our market” — but that is a 101-level mistake. Focusing on one person’s understanding of their problem isn’t constraining your solution’s appeal. In fact, it is the opposite.
The movie Objectified explains how IDEO designed an accessible and highly popular toothbrush by focusing specifically on the needs of small children learning to brush, rather than “all people with teeth.” Alan Cooper went even further when he designed the program Plan*It with just one person in mind — someone he actually met and spoke to.
Compared with the incredible value of things customers have actually said, things that sound like customers might say them are worthless. And yet that’s exactly what LLMs do — produce text that appears plausible. An LLM can’t talk about the last time it had to accomplish a task. It’s impossible to drill-down to understand how the use of a product intersects with its context because it has no lived experience. Unlike a human being, varying your prompting will never reveal anything that isn’t already in the model — the insight that separates products from commodities. All the model can do is put those words in a synthetic mouth.
And there is one more very important difference between an LLM and a customer:
The LLM can’t buy your product.
No, AI user research is not “better than nothing”—it’s much worse was originally published in UX Collective on Medium, where people are continuing the conversation by highlighting and responding to this story.
Leave a Reply