What were the discussions and suggestions proposed by our community in the last five years?
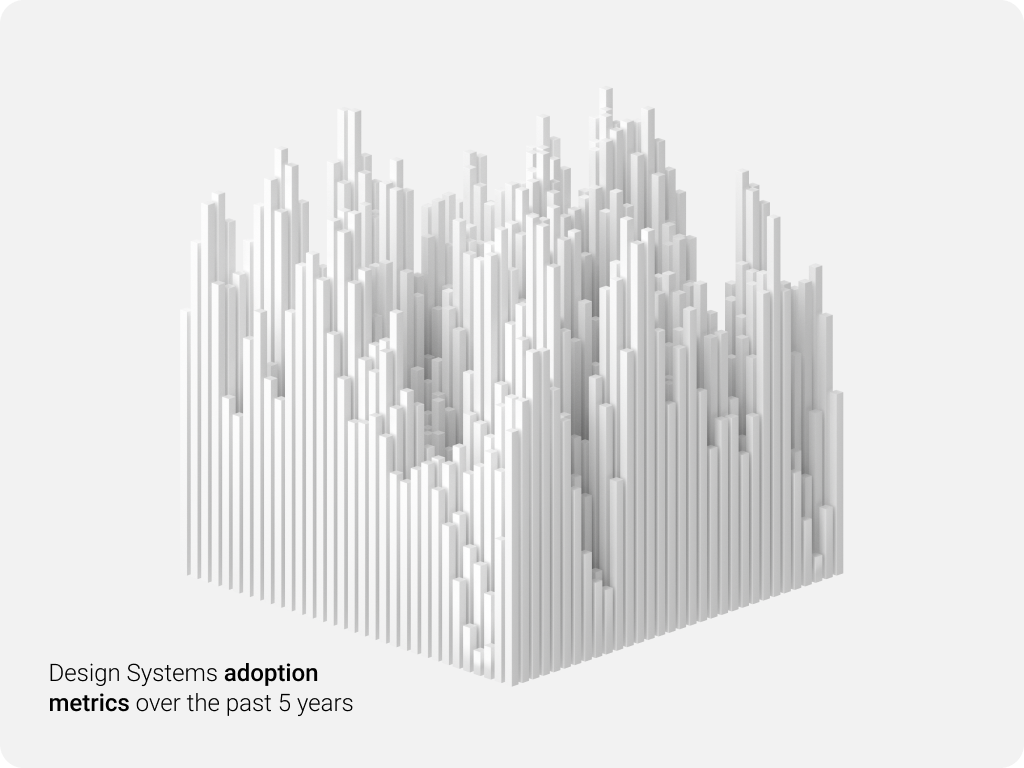
Imagine that you and your team have finally managed to build a Design System (DS) to be applied across various products. However, even after going through this process, you’ve noticed several different impediments affecting the adoption levels of the DS within your teams — both in relation to the designers and developers involved.
This scenario is not uncommon. In fact, it is considered one of the most challenging stages in the production of a Design System — as seen in the report by Sparkbox (2019), where 52% of respondents reported adoption as their biggest evolution challenge. That’s why, to overcome the DS implementation stage, there needs to be some research and measurement process regarding product adoption.
It was due to this pain point that I brought this article to life about adoption metrics in Design Systems. Since it’s a complex subject, I wanted to gather as many perspectives as possible, bringing everything that has been discussed on the topic in the last five years! This is a challenge because, despite the existence of materials on the topic, each person speaks in their own language, where clear definitions of the metrics used are sometimes lacking.
Therefore, this writing aims to provide a synthesis, creating discussions on the subject, groups of possible metrics for use, and suggestions for numerous measurement tools. Come with me!
Sections
1. Methodology
2. Overview and Discussion
3. Qualitative Metrics
4. Quantitative Metrics
5. Tools for Measurement
1. Methodology
Before delving into the research itself, let’s take a look at how this extensive mapping over the last five years was conducted.
If you prefer to skip this section for now, feel free to move on to the next without any issues. However, if you are interested in this part, feel free to explore the step-by-step process of the research, including the data generated and analyzed in tables.
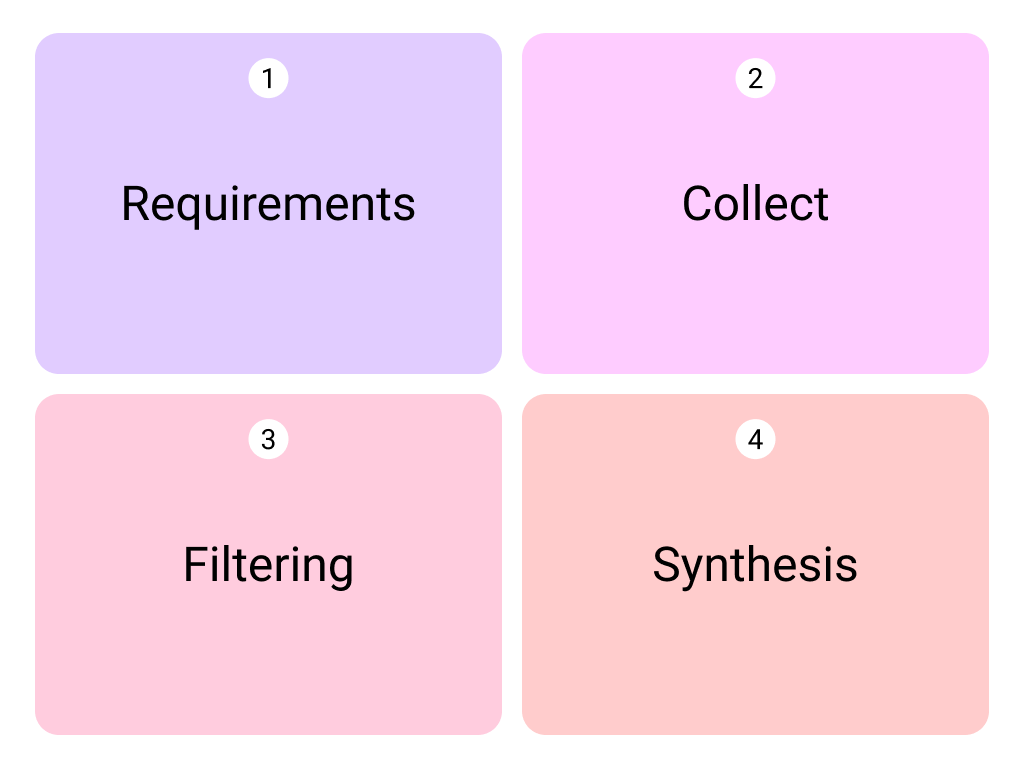
Everything you can see in this table was generated through a systematic review¹ of articles and reports published on Design Systems adoption metrics with two requirements:
1) The production had to be published in the last five years (data collection occurred until November 2023).
2) The content had to be written in English.
Here are some more details about this five-year review. Non-academic writings published on the internet in spaces designated for communication were collected from the beginning. The requirement was that these materials had to be free to create knowledge without access restrictions. To achieve this, searches were conducted on three platforms: Google, Medium, and LinkedIn. However, only a systematic search on Google proved sufficient to collect the rest of the articles published in other spaces.
For the search, “design system adoption metrics” and “design system metrics” were used as terms to aggregate everything that was found. After this extensive process, the data filtering was initiated based on some inclusion and exclusion criteria for the collected links:
Inclusion criteria: Articles or reports that discuss metrics to assess the levels of adoption of a Design System.
Exclusion criteria: Materials that deviate from the theme “DS adoption metrics.” Examples of these cases include writings that discuss maturation without mentioning metrics, articles that talk about DS quality metrics instead of adoption, and discussions revolving around adoption without mentioning any type of metric. Any materials in other media, such as videos, were also excluded.
Is it clear how this entire review was conducted with care to ensure that no aspect of this subject was overlooked? Now, let’s explore everything that was found.
2. Overview and discussion
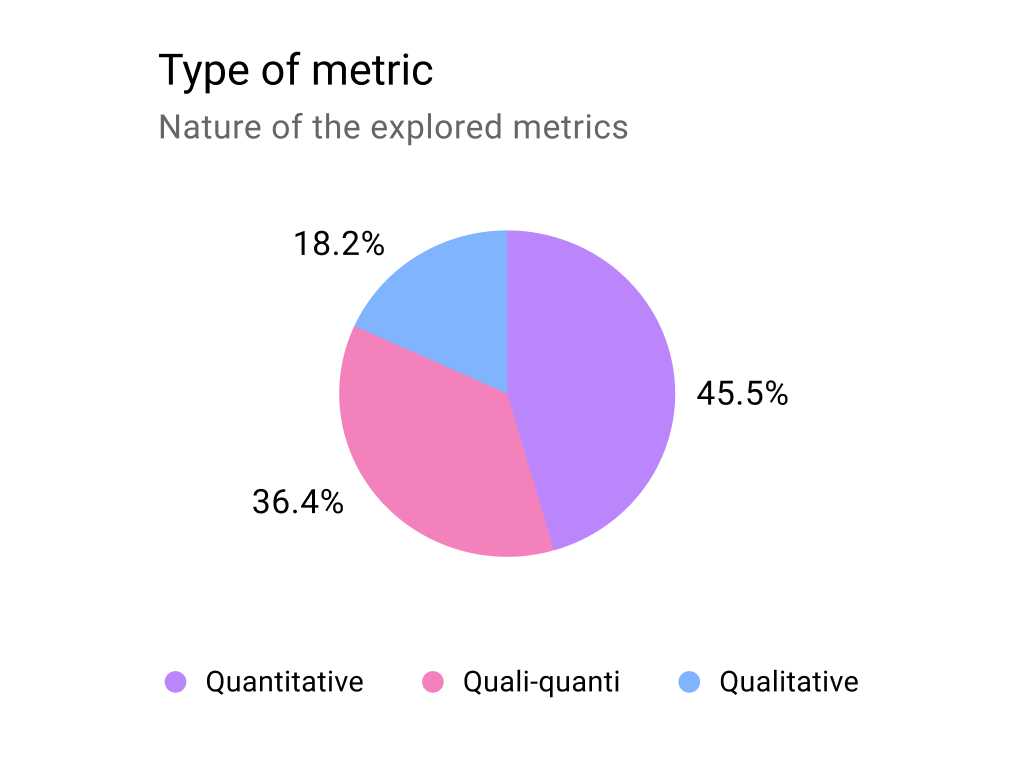
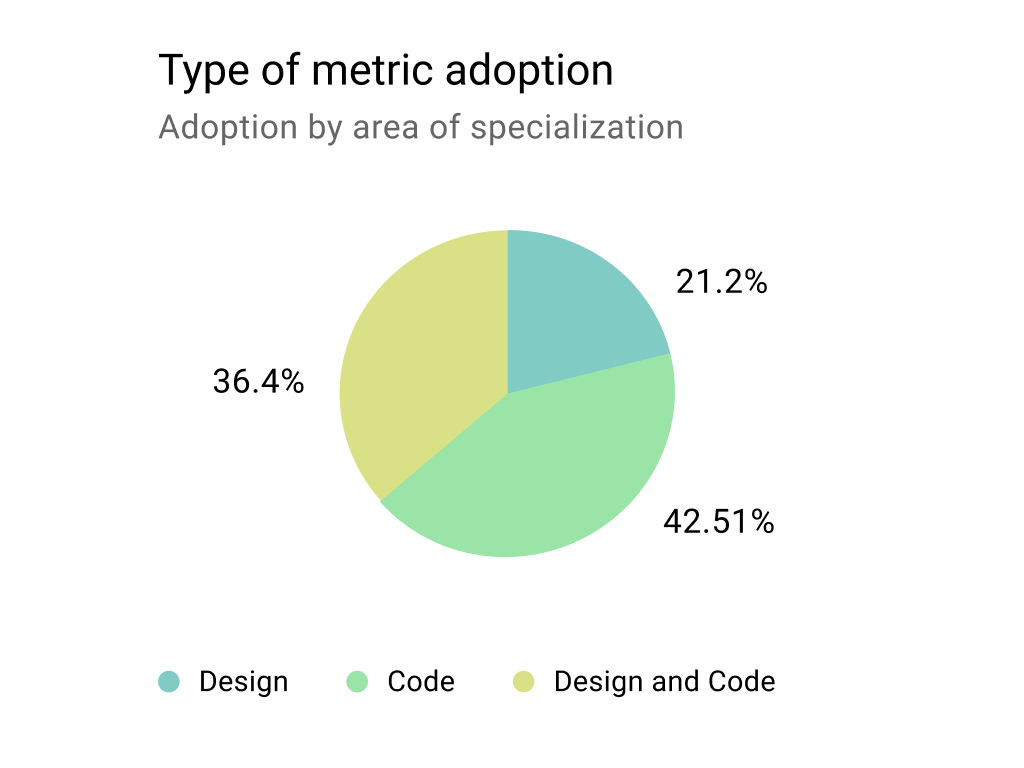
In the last half-decade, there has been an interesting division in the materials created. Clearly, there is a higher percentage of debate around quantitative data (or mixed, but often privileging quantitative data) and measurement done at the development level only. However, there have also been many discussions around the idea of measuring adoption as a human factor — totaling nine articles that engaged in a critical debate around the culture of quantification.
Cristiano Rastelli (2019), a designer who worked on Cosmos, the Design System of Badoo, states that despite understanding the importance of adoption metrics, he exercises caution regarding the need to measure adoption quantitatively to prove its existence. He argues that relying solely on metrics to make decisions — without considering our minds, hearts, and sometimes intuition — can be a double-edged sword.
Robin Cannon (2022), one of the creators and leaders of the IBM Carbon Design System, emphasizes that adoption numbers can sometimes turn into vanity metrics. He notes that high adoption rates can be based on authoritative management decisions, resulting in no tangible benefits for product users or working teams. This view strengthens Jonathan Healy’s (2020) argument from Morningstar DS, emphasizing that adoption metrics alone are not indicative of a quality product. Therefore, it is essential to incorporate qualitative assessments from Product Design teams for a more empirically comprehensive analysis.
The debate over the past years shows that if we are to measure adoption, we need to understand the how and why. Dan Mall (2023), the founder of Design System University, argues that adoption as a metric is a “lagging” indicator — meaning it can only be measured when there is already some use of the DS. Therefore, using these metrics can be a mistake in situations where the use of the Design System was not the most strategic choice for a particular product — such as cases where the components are not sufficient for building the desired interface.
For Dan, the success of a DS does not simply lie in its widespread unrestricted adoption across all products. On the contrary, true success lies in the effective organization of work processes. In other words, the most important thing for him is to measure the level of presence of the team responsible for the DS in the ideation phases of a product. This way, the measurement of adoption becomes more accurate when it is evident that the use of the Design System is the most strategic decision from the beginning of the project.
Therefore, the most important point of this debate is summarized by Forrester (2021) in a blog post for Adobe: if you choose a specific metric, try not to measure adoption in isolation. Measuring adoption is important to know if teams are using the created product, but even more important is establishing a central goal for what you aim to achieve with the tool! While one team’s goal may be to increase production speed, another team’s goal may be primarily to increase product accessibility levels.
In a correlated manner, Mandy Kendall (2020) from Sparkbox emphasized the importance of not creating simplifications through metric isolation. In her article, she argues that creating quality metrics can have a significant impact on adoption within the team, as it demonstrates the level of care taken in creating DS components. To generate and measure adoption, she suggests creating visual cards in the product documentation, measuring usability, accessibility, code quality, and performance levels for each component.
Thus, it is possible to track the increase in adoption per component as their quality levels rise — work that is done through versioning by component rather than library versioning. In a slightly different approach, Ellis Capon (2021) from NewsKit argues the same, advocating for the use of Accessibility Score and Core Web Vitals Scores: if they increase, there is a high probability of greater Design System adoption. It all depends on the application’s goal.
3. Qualitative Metrics
From everything discussed so far, it is evident that in the past five years, there has been a recognition that qualitative measurement needs space, as even a Design System needs to be user-centered. Many specific types of qualitative metrics have been introduced, but we can synthesize them into a broad category.
Satisfaction Level
Exactly 50% of the articles address the importance of measuring satisfaction qualitatively. Although a significant portion talks about NPS (Net Promoter Score) and SUS (System Usability Scale) as methodologies to generate this metric, almost all emphasize the importance of going beyond the classic survey.
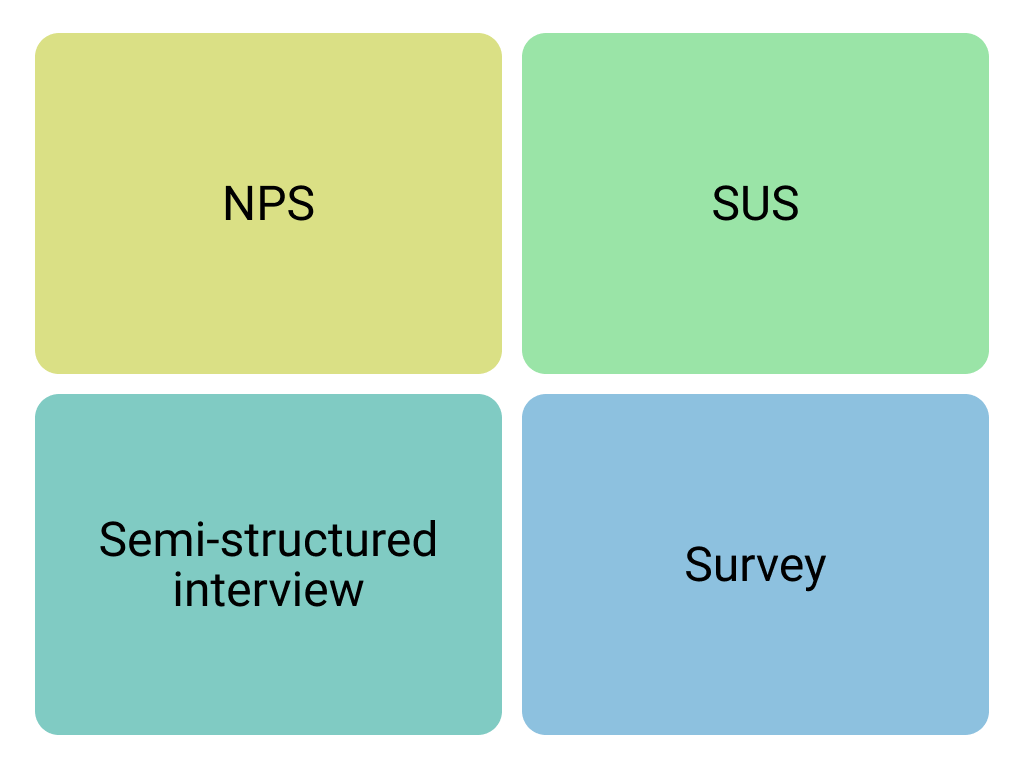
Brooke Rhees (2023) at Lucidchart demonstrated this by discussing how this qualitative measurement of success can be done from an engagement perspective. During research with teams, they recorded people’s emotions to understand if there was enthusiasm about the application of the Design System. Therefore, this type of research not only intends to create data but also aims to generate a sense of ownership and community among teams using the product. This management and exchange concern is something I found in related articles, such as the one about the reconstruction of Spotify’s Design System.
During these in-depth exchanges, specific points within the satisfaction level can be measured through this engagement and ownership perspective, such as:
- Satisfaction with the UI of the components
- List of components considered necessary for the Design System
- Level of ease and convenience in using the Design System
- Understanding level of documentation on best usage practices
- Feedback with improvement points for any aspect of the Design System
4. Quantitative Metrics
Well, all this discussion does not diminish the importance of metrics for generating quantitative data. On the contrary, it highlights the need to carefully choose a metric to shed light on any issues impacting the adoption of a Design System.
Here, you will find metrics that synthesize various specific calculations I found in the articles — including measurement methods in both design and code.
4.1 Component Adoption
Measures the adoption of a Design System through the use of its components. It is a good metric to gauge whether the components are considered sufficient for building interfaces. This is the most common type of adoption metric.
Possible metric calculations
- Number of components inserted (overall or specific per component)
- Number of components with detached instances (overall or specific per component)
- Number of new components outside the DS in projects (also referred to as homebrew in some articles)
- Most/least used components
- Any of the above filtered by product, team, or client
Components in Design
Regarding Pinterest’s DS, Lingineni (2023) talks about the importance of measuring “design adoption” — that is, measuring the use of components in design before implementation in code. This metric indicates, in a measurable way, that there will be a return on investment in the development phase.
When the team measures adoption in design, calculations are based only on files used in the last two weeks marked as “handoff.” This reduces the noise from the quantity of existing files (such as “draft” files) to see what is currently happening with the teams at the time of measurement. This measurement is primarily done through the calculation:
DS Component Adoption % = Nodes originating from the DS / All nodes
This calculation is suggested as a better way to measure than simply quantifying component insertions, allowing visualization of the DS usage coverage in a specific project.
Data Visualization
One of the best ways to visualize this metric was shown at the 2022 React Summit in an analysis of the Graphana UI. The suggested data visualization is as follows:
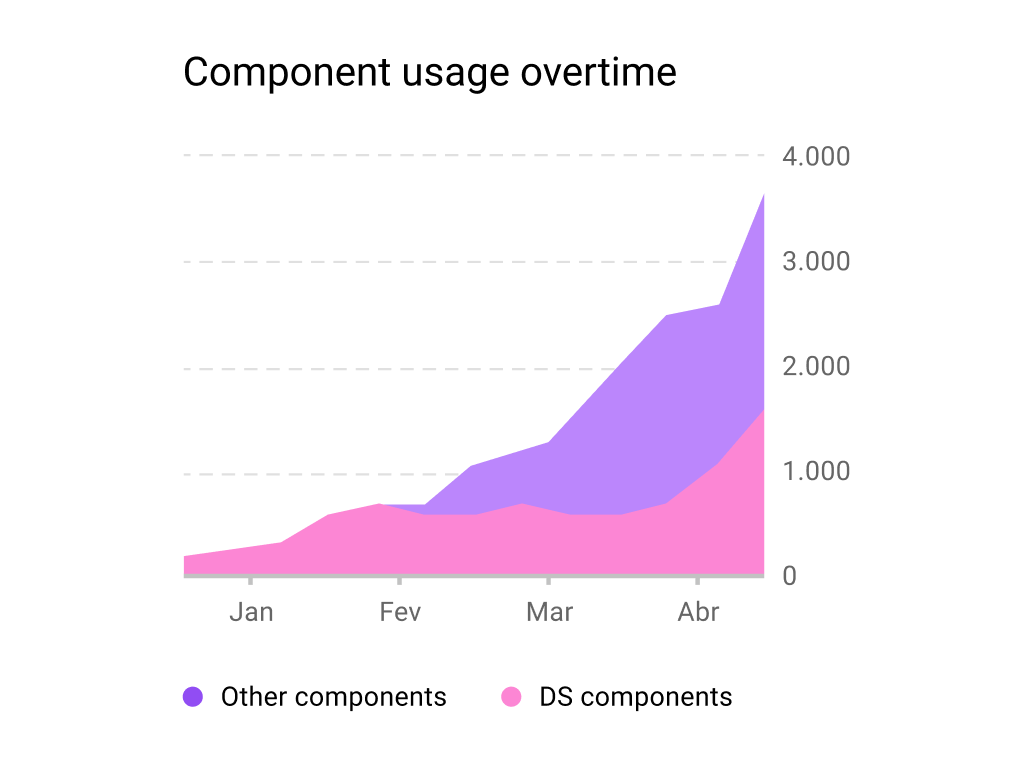
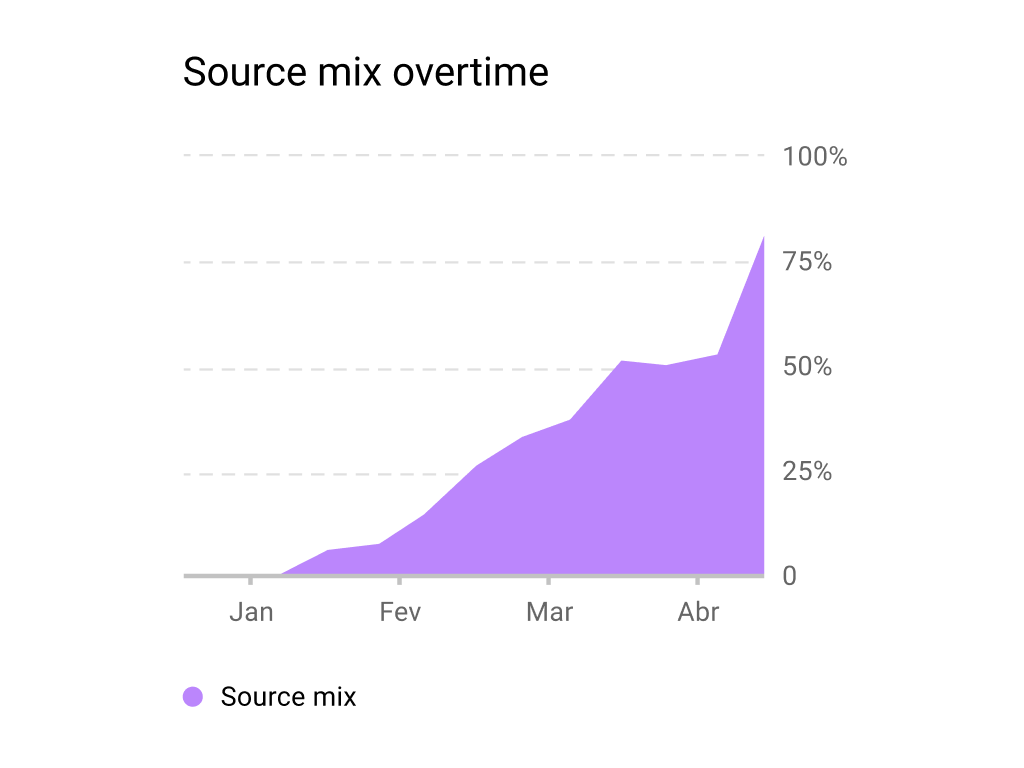
In addition to allowing visibility into adoption over time, there are two possibilities for visualization: by quantity or by percentage (referred to as source mix). The percentage chart is seen as preferable for a relative view that is independent of numerical changes that may occur across projects. This was also suggested by Veriff (2019), highlighting the importance of this consideration.
Moreover, Steve Dennis (2022) — a text explored in an article from Zero Height — emphasizes that counting the number of times a component is used can be challenging in some cases. When a button in the header of an application appears on 30 pages, should it be counted as 30 used buttons or a single button?
Because of this, Dennis proposes measuring how many products have implemented a component at least once in their source code. This approach allows the identification of components in use, highlights products that make intensive or minimal use of the design system, and provides a more accurate view of adoption.
Measurement in Code
- Screen Visualization: Some articles explore the idea of creating copies of projects and modifying components to visually see everything coming from the Design System (like adding a striking stroke). You can learn more about this in Danisko’s article (2021), where the use of visualization is suggested as a secondary metric to identify areas for improving interfaces.
- IDs or Props: Some texts suggest adding IDs to components to ensure the unique identification of DS elements to generate data analysis. When React is used in code, some articles suggest tracking specific props used in components.
4.2 Token Adoption
Measures the adoption of a Design System through the use of its tokens. It is a good metric to measure less visible applications than components.
Possible metric calculations
- Number of text tokens
- Number of texts without tokenized styling
- Number of color tokens
- Number of non-tokenized hex codes
- Any of the above filtered by product, team, or client
Data Visualization
When thinking about token adoption, it becomes apparent that it is indeed similar to component adoption. Therefore, most articles mentioning it return similar adoption calculations, suggesting that the best use is percentage visualization, possibly in an overtime graph. To measure the adoption of typography, for example, the following calculation is suggested:
DS Typography Adoption % = DS Text Tokens / All texts
4.3 Library or Version Adoption
Measures the adoption of a Design System through the use of its libraries. It is a good metric for gauging the adoption of significant parts of the DS and its versioning.
Possible metric calculations
- Number of uses of a specific DS library
- Number of uses per version of the libraries
- Number of uses per version of the DS
- Any of the above filtered by product, team, or client
Data visualization
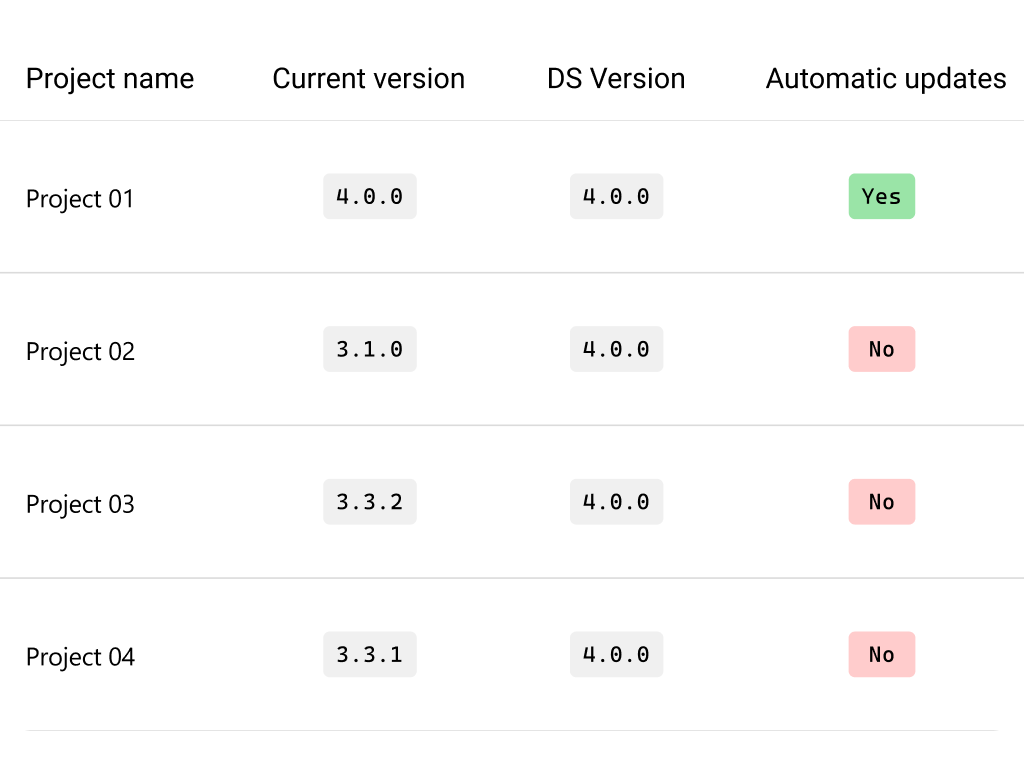
Despite the metric above focusing on measuring the quantity of uses of specific libraries (such as the number of uses of an icon library, for example), the more critical reflection on adoption relates to the issue of versioning.
One of the significant challenges in Design Systems adoption arises due to the evolution of the product over time. Therefore, it is quite common for projects to use older versions, necessitating control over the migration processes to the most updated version of the DS. Articles like those from Morningstar (2020) suggest version control tables for projects, encouraging automation of updates whenever possible (with the ability to record whether there is automatic updating in the code).
However, I want to remind you that the issue of versioning data depends a lot on the structure of your DS! The article I mentioned above focuses on versioning by component instead of library versioning. Therefore, your data table could also be structured in the following way.
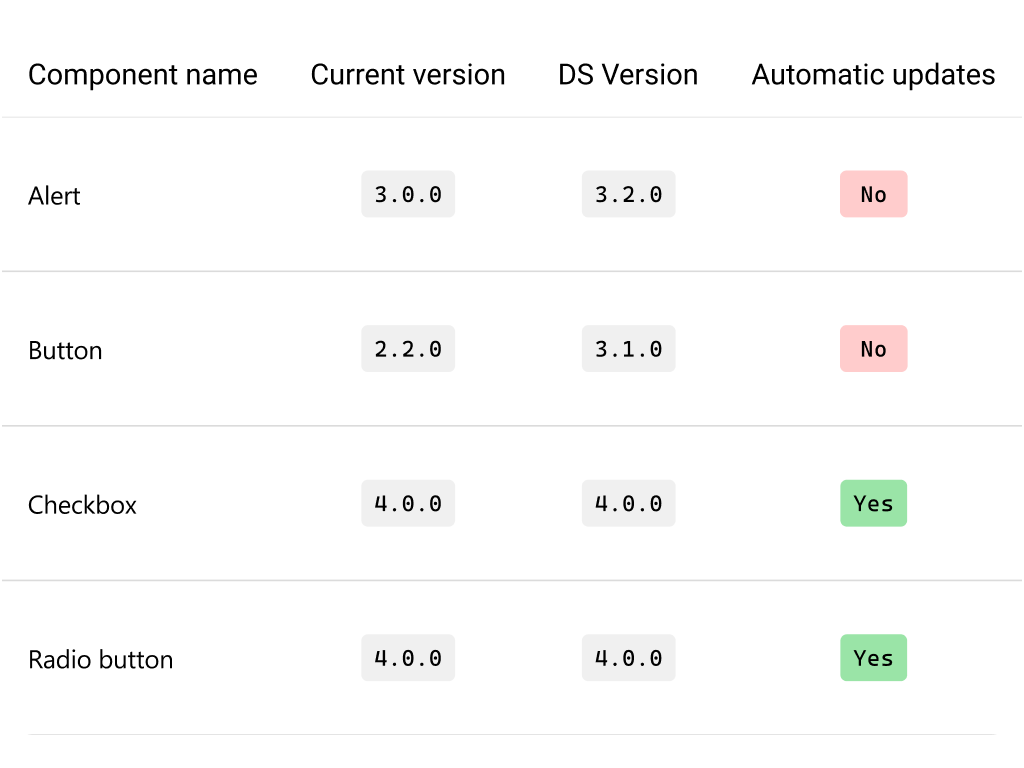
4.4 Adoption by Access
Measures the adoption of a Design System through access to its files and libraries. It is a good metric to gauge whether the complete files containing usage documentation are being utilized.
Possible metric calculations
- Number of visits to files/libraries
- Number of users of files/libraries
- Access frequency
- Most visited pages
- Time spent on pages
- Number of comments on the file
- Number of link shares
- Any of the above filtered by product, team, or client
4.5 Adoption by Time Savings
Measures the adoption of a Design System through production speed. It is a metric that goes beyond adoption, often referred to as “production efficiency” or “production speed.” It is a good metric to demonstrate the value of a DS when comparing before and after.
Possible metric calculations
- Average time to design a single component, screen, or complete prototype (also called “development speed”)
- Hours per month dedicated to designing new components per designer
- Quality Assurance (QA) Work Time
- Number of changes made by a developer (commit dates, the number of files changed, and especially the number of lines of code added or removed with each change)
- Any of the above filtered by product, team, or client
Data Visualization
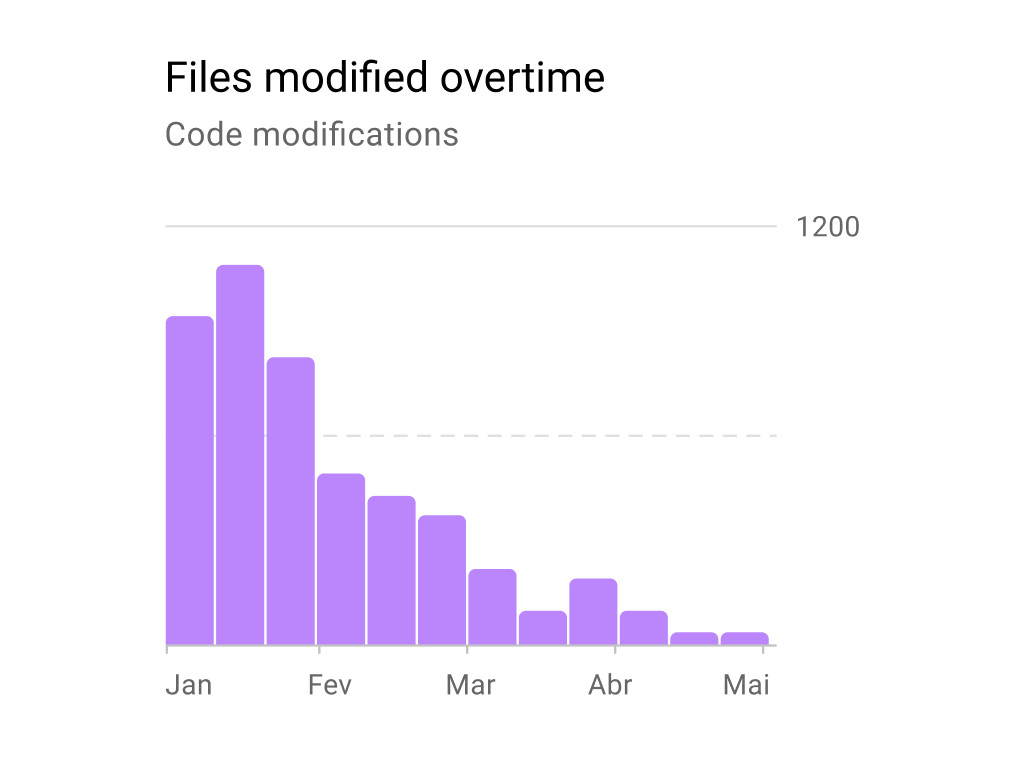
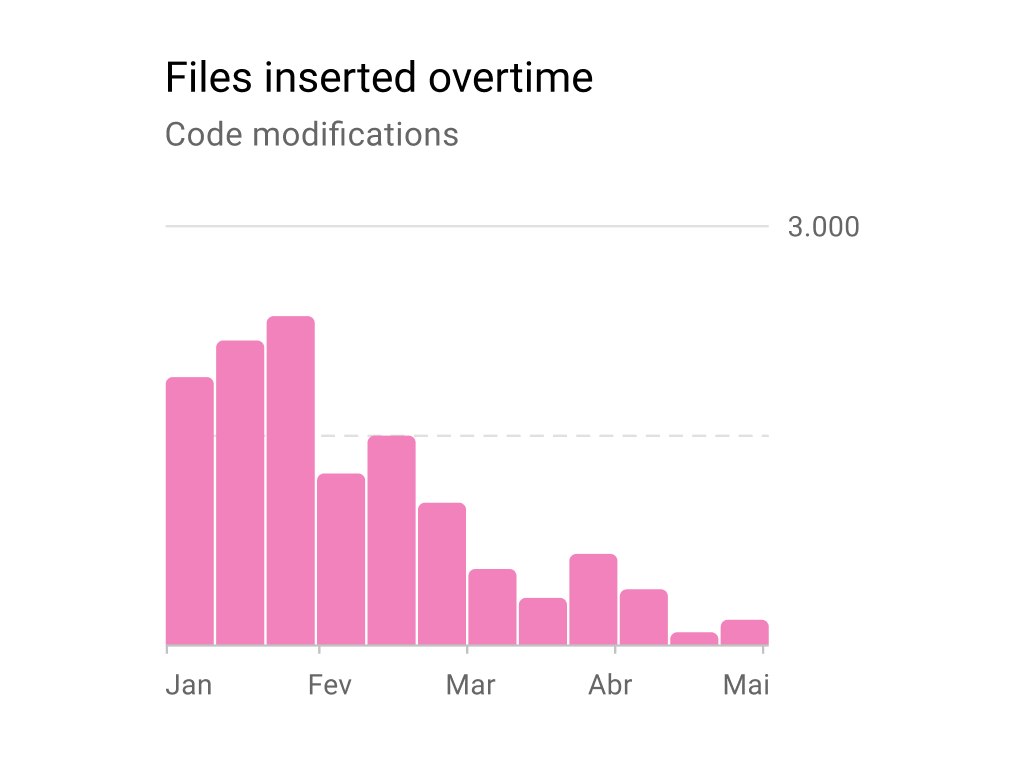
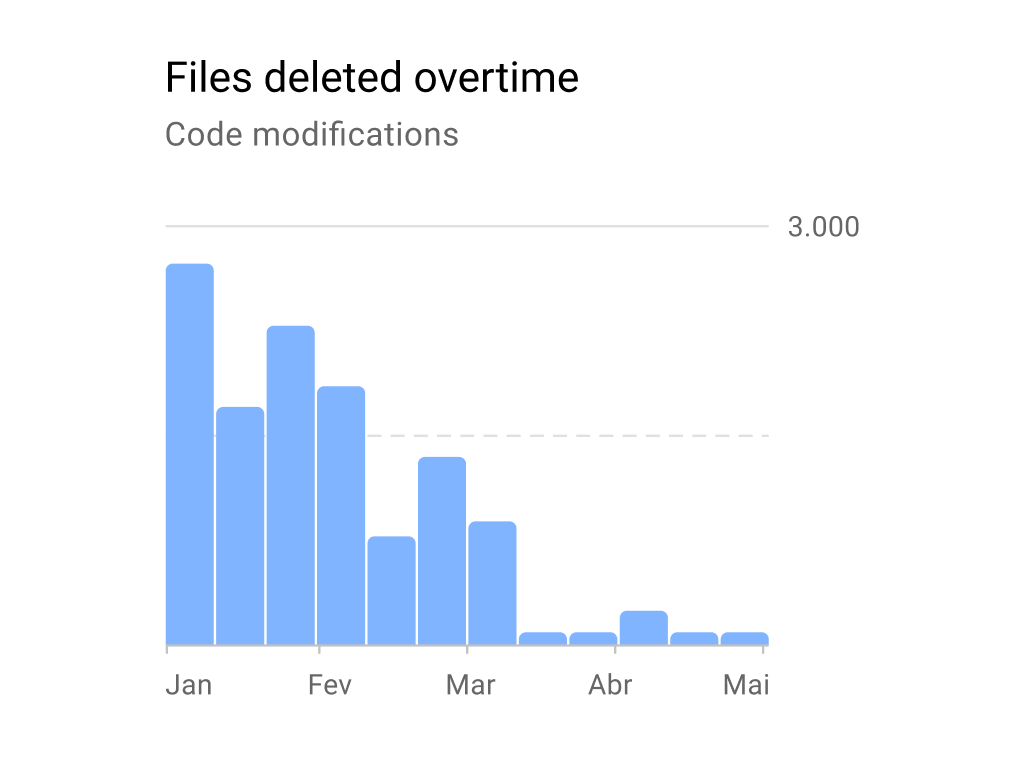
Generating and visualizing data about work time is always complex. After all, how do you measure this accurately?
One of the articles that synthesizes this in the best way is Rastelli’s (2019), which creates histograms to visualize changes in project code over time. This allows you to see if there is indeed a reduction in the amount of work for developers after the implementation of the Design System: if yes, the histogram should show the reduction in inserted, modified, or deleted files.
4.6 Adoption by Production Cost Reduction
Definition: Measures the adoption of a Design System through the reduction of production costs. It is a metric related to the reduction of production time, often referred to as the Return on Investment (ROI) of a Design System.
Possible metric calculations
- Annual savings
- Average cost per hour of design/development
Data Visualization
According to Ryan Lumm’s article (2019), we can estimate the costs saved with a simple formula:
Annual Savings = Hours per week x 52 weeks x Hourly Rate x Team Size
For example, if a designer spends approximately 2.5 hours creating new components from scratch per 52 weeks (work weeks in a year) with an hourly rate of $70/hour, the company would save $9,100/year. The formula is easily adaptable for monthly or semester visualization — just modify the number of weeks!
5. Tools for Metrics
In addition to choosing which metrics to use, the articles mention various tools that can be used to measure the adoption of prototypes created by designers and the code produced by developers. Here is a compilation of these mentioned tools.
Specific Tools
1. Figma Library Analytics — Mentioned in 24% of the texts. The library analytics enable optimizing component usage, identifying the most used components, detecting frequent detaches, recognizing underutilization, analyzing team engagement, and comparing usage between libraries, as well as finding examples to improve documentation.
2. React Scanner — Mentioned in 16% of the texts. React Scanner is a tool that analyzes React code, extracting information about the usage of components and props. It traverses the provided directory, compiles a list of files to be analyzed, and generates a JSON report. This report can be used to assess the frequency of using specific components, props, and the distribution of prop values in specific components.
3. Segmentio Dependency Report — Mentioned in 16% of the texts. The Evergreen team provided detailed insights into creating adoption data dashboards using this tool they created, which is open source. It is an interesting tool that generates interactive charts (such as treemaps), with a thorough explanation in Ransijn’s article (2018).
4. Radius Tracker — Mentioned in 8% of the texts. Radius Tracker generates reports measuring the adoption of the design system in code, calculated from the bottom up based on the usage statistics of individual components automatically collected in your organization’s repositories.
5. Octokit— Mentioned in 8% of the texts. It was used to track which organizations have a package.json file or Node.js code. It allows the generation of detailed reports, providing insights into which organizations and projects are implementing a specific DS.
6. Custom Style Script— Mentioned in 4% of the texts. A plugin that can be used for a visual measurement of component usage, highlighting changes in CSS.
7. GitHub Actions — Mentioned in 4% of the texts. It is a continuous integration and delivery (CI/CD) platform. It allows automating the workflow, building, testing, and deploying code directly from GitHub.
General Tools for Data
1. Metabase — Cited by Stanislav (2023) and Sarin (2023).
2. Google Analytics — Cited by Jules Mahe (2023).
3. D3.js — Cited by Stanislav (2023) and Rastelli (2019).
4. Hotjar — Cited by Jules Mahe (2023).
5. Prisma — Cited by Sarin (2023).
6. Mixpanel — Cited by Stanislav (2023).
7. Tableau — Cited by Stanislav (2023).
Liked this extensive mapping? Feel free to contact me through my LinkedIn and provide feedback — whether positive or negative!
¹ Systematic Literature Review is a scientific methodology used in many fields of knowledge. It is usually applied to academic texts of a scientific nature, but I adapted this methodology to conduct a review of technical texts on Design Systems.
References
Certamente, aqui estão os links sem hiperlinks:
CANNON, R. (2022). Design system adoption numbers… just a vanity metric? Available at: https://shinytoyrobots.substack.com/p/design-system-adoption-numbersjust
CAPON, E. (2021). Evolution of design system KPIs. NewsKit design system, Medium. Available at: https://medium.com/newskit-design-system/evolution-of-design-system-kpis-490f87097473
DANISKO, F. (2021). How we measure adoption of a design system at Productboard. ProductBoard. Available at: https://www.productboard.com/blog/how-we-measure-adoption-of-a-design-system-at-productboard/
DANNIS, S. (2023). How to Measure Design System Adoption: automated tracking of token and component adoption. UX Collective — Medium. Available at: https://uxdesign.cc/how-to-measure-design-system-adoption-a17d7e6d57f7
FORRESTER. (2021). Best practices to scale design with Design Systems. Adobe. Available at: https://blog.adobe.com/en/publish/2021/05/26/best-practices-to-scale-design-with-design-systems
GALVAO, T. F., & PEREIRA, M. G. (2014). Revisões sistemáticas da literatura: passos para sua elaboração. Epidemiol. Serv. Saúde, Brasília, 23(1), 183–184. Available at: http://scielo.iec.gov.br/scielo.php?script=sci_arttext&pid=S1679-49742014000100018&lng=pt&nrm=iso
ISBER, S. (2021). Insights & Metrics that Inform the Paste Design System. Twilio. Available at: https://www.twilio.com/blog/insights-metrics-inform-paste-design-system
KENDALL, M. (2020). Using Scorecards to Encourage Adoption of Design Systems. Sparkbox. Available at: https://sparkbox.com/foundry/versioning_design_systems_components_with_component_scorecards
LINGINENI, R. (2023). How Pinterest’s design systems team measures adoption. Figma. Available at: https://www.figma.com/blog/how-pinterests-design-systems-team-measures-adoption/
LUM, R. (2019). How to: measure a Design System’s impact. UX Collective — Medium. Available at: https://uxdesign.cc/how-to-measure-design-system-impact-guide-f1f9f0c3704f
MAHE, J. (2023). How to measure the dev side of a design system. ZeroHeight. Available at: https://zeroheight.com/help/guides/how-to-measure-the-dev-side-of-a-design-system/
MALL, D. (2023). In Search of a Better Design System Metric than Adoption. Dan Mall. Available at: https://danmall.com/posts/in-search-of-a-better-design-system-metric-than-adoption/
MEIUCA. (2022). Design System e OPS: Mostra tua Cara, V2. Available at: https://report.meiuca.co/
RANSIJN, J. (2018). How We Drove Adoption of Our Design System. Twilio Segment Blog. Available at: https://segment.com/blog/driving-adoption-of-a-design-system/
RASTELLI, C. (2019). Measuring the Impact of a Design System. Medium. Available at: https://didoo.medium.com/measuring-the-impact-of-a-design-system-7f925af090f7
RHEES, B. (2023). Learning how to measure a design system’s success. Lucidchart. Available at: https://www.lucidchart.com/techblog/2023/01/09/learning-how-to-measure-a-design-systems-success/
SARIN, P. (2023). How to track Design System Adoption. Brevo. Available at: https://engineering.brevo.com/how-to-track-design-system-adoption/
SMOOGLY, A. (2022). Find Out If Your Design System Is Better Than Nothing. GitNation. Available at: https://portal.gitnation.org/contents/find-out-if-your-design-system-is-better-than-nothing
SPARKBOX. (2019). Design Systems Survey. Available at: https://designsystemsurvey.seesparkbox.com/2019/#section-2
STANISLAV, K. (2023). How can the efficiency of design system be measured? UX Planet — Medium. Available at: https://uxplanet.org/how-can-the-efficiency-of-design-system-be-measured-9531f27ce338
The challenge of Design Systems adoption metrics was originally published in UX Collective on Medium, where people are continuing the conversation by highlighting and responding to this story.
Leave a Reply