Be opinionated, embrace criticism, and improve the arguments of others.
Taking a deeper look at how OKRs influence strategic decision-making.
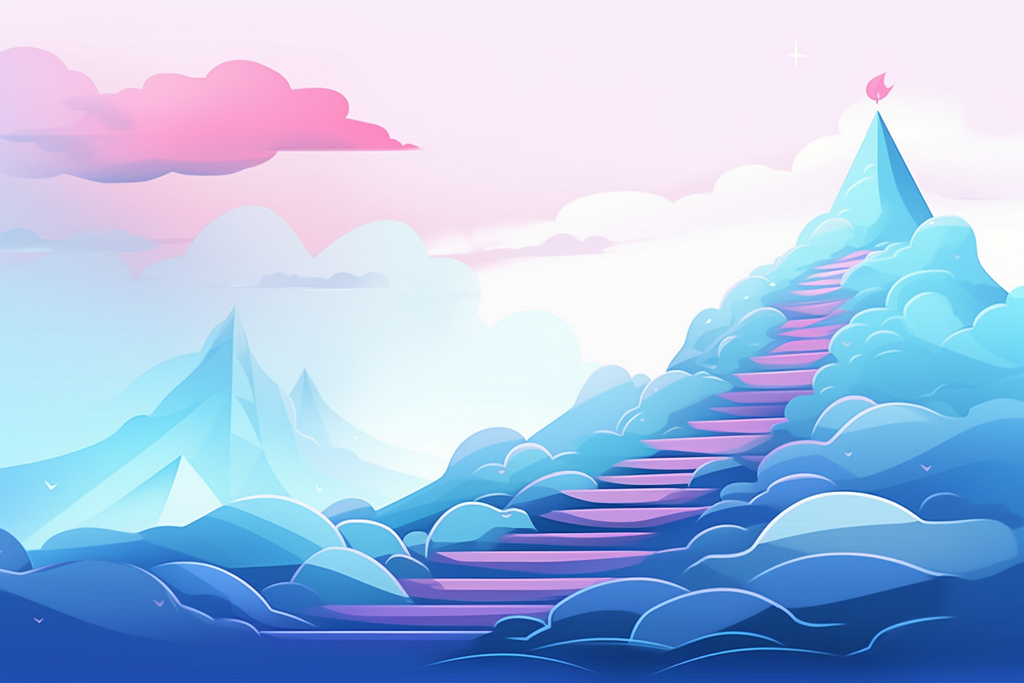
Before the pitchforks come out, I’m a fan of OKRs — I’ve seen their implementation transform companies for the better, and I’ve personally experienced their challenges. As with anything that gains seemingly unfettered support, it’s worth investing time in understanding the counter-arguments.
If you’re new to OKRs or are not yet familiar with the framework, my personal favorite subject matter experts are Jeff Gothelf & Felipe Castro. And of course, whatmatters.com is also a great place to start.
This post is split into a series of different arguments. They tie together thematically, but it’s just as easy to jump around. Feel free to jump to topics that interest you:
- Intro: My personal experience with OKRs
- Intro: The surface problems
- 1. Causal rationality vs. effectual reasoning
- 2. The explore-exploit dilemma, is something missing?
- 3. Goal-induced Blindness
- 4. Escalation of commitment
- 5. Distorted incentives and risk preferences
My personal experience with OKRs
I’ve been around OKRs (a goal-setting framework developed by Andy Grove at Intel and made popular by John Doerr at Google) since before Doerr published Measure What Matters in 2018 — both in using them with my own teams and designing OKR tooling for enterprise customers at Atlassian.
I remember being introduced to OKRs sometime in late 2017 or early 2018 through working with teams at Tableau and Eventbrite — they were anchoring their strategic planning meetings around this framework and it’s the first time I saw it in action. There were two things that stood out to me (and I think many have had the same experience):
- Teams were having ‘different’ outcome-driven conversations. At the time, everyone was talking about teams ‘knowing the why’ behind work, but the conversations I saw happen around OKRs were the first experiences where I actually saw that happen.
- Decision making and ‘Solutioning’ was decentralized. OKRs created a new type of language for progress — we saw cascading trees of epic/feature/story progress bars traded out for outcome-oriented dashboards. They quickly created an environment of trust just by agreeing on what success looked like.
Looking back it was a watershed moment — a framework that actually produced real results (and quite quickly). It’s accessible, powerful, and like any other framework, it can be implemented ineffectively, but its simplicity is one of its best features. It’s a way to incorporate outcome-focused principles right out of the box.
A few months ago, I wrote a post on exploring goal setting and OKRs through the lens of decision making, but this time, we’re digging into some of the potential pitfalls.
And though I am a supporter of OKRs, I’ll take a critical/skeptical perspective for the sake of this post 😈
The surface problems
When we look at OKRs through the lens of decision making, I think there are some clear problems (already known and felt by most) that can inhibit decision making. They surface situations that make decision making murky and difficult.
Specifically, these include:
- Correlation and Causality: How do we know that what we’re doing is actually the thing that’s moving the needle?
- Leading vs Lagging Indicators: What if the initiatives that look like they aren’t working just aren’t working yet?
- Escalation of Commitment: What if we find out that what we’re chasing is wrong in the first place? We tend to hold on to losing bets.
There are behavioral challenges as well — like ‘set & forget’, poorly defined objectives, and structuring KRs as outputs — but I would argue these problems still plague OKRs even if teams are doing everything ‘right’.
The difficulty with these problems is that they are rooted in human behavior — and in my opinion, tools aren’t all that great at improving human behavior, only augmenting and improving the effectiveness of existing behavior (or forcing behavior — which isn’t improving; it’s introducing friction and stress until something breaks).
If we’re being honest, in most cases, we’re relying on human judgment to correct these issues and each one comes with behavioral traps that we are individually blind to:
- The trap of causality illusions, illusory correlation, and the representativeness heuristic — we tend to see correlation, causation, and ‘meaning’ in data that isn’t there.
- The trap of optimism bias, pessimism bias, and strategic misrepresentation — we tend to misinterpret or overexaggerate signals of success/failure and manipulate information in response to incentives.
- The trap of status quo bias, sunk cost fallacy, and commitment bias — we tend to entrench ourselves in existing pursuits/investments even in the face of overwhelming contradictory evidence.
These traps have little impact on how effective or well-crafted our goals and measures are, but they do impact our ability to achieve those goals. OKRs, in isolation, do not improve our ability to make better assumptions or improve/check human judgment — they provide helpful constraints for decision making (e.g. it’s clear we are trying to achieve x, not y), but they don’t have any effect on our ability to achieve the goal.
OKRs are a tremendous improvement on traditional output-driven project planning, but they weren’t designed as a substitute for strategy — our current situation is the result of the choices we make in our competitive environment, not based on whether or not we crafted the right goals (or even achieved them).
The goals themselves are a choice.
This is an intuitive conclusion — two different teams/companies can have the same goal with different capacities to achieve that goal. There are limits to the competitive advantage of ‘crafting better goals’ — and competition in this environment is relative, not absolute. It’s an infinite game.
“Desire (as with hope) is simply not a strategy. The desire to achieve the named key results won’t cause those key results to happen. You may desire the substantial rise in your NPS, but if you are serving customers that your key competitor serves better than you do, your NPS is unlikely to rise — even though you really want it to.”
Roger Martin, Stop Letting OKRs Masquerade as Strategy
1. Causal rationality vs. effectual reasoning
The argument: Starting with known ends (goal-setting) is effective for causal thinking — and for what Sarasvathy describes as ‘creative’ causal thinking, but may not be as effective in scenarios that require ‘effectual reasoning’.
There are two problems that have interested me recently that were not initially obvious to me — and I think it explains why OKRs, alongside all of their positive features, don’t feel as effective in low-validity, complex domains.
- In navigating uncertainty, particularly in ways that produce asymmetric results, we typically do not start with a ‘known end’ — which is why asymmetric results are obvious in hindsight, but ‘unimaginable’ at the decision point.
- We know that one of OKR’s most powerful features, focus, may have unintended consequences on decision making in these environments.
As I mentioned, I am an advocate for OKRs, but we shouldn’t forget that the goal itself is a choice and a constraint. This is of course a feature, not a bug, but we can’t ignore that the constraint has a profound impact on our decision making.
The choice of ‘what the goal should be’ and ‘how to measure it’ boxes us into a ‘Known End, Unknown Means’ scenario. This means we know the desired outcome or change in behavior (the end), but the challenge is identifying the most effective means to achieve that end.
We can assume the alternative scenarios as well:
- Known End, Known Means: We know what we need to do and how to do it — a superior plan and the ability to execute that plan is a competitive advantage here.
- Unknown End, Unknown Means: Exploration for the sake of exploration — this is where inventions like the microwave, penicillin, and Velcro come from. Curiosity and accidents.
- Unknown End, Known Means: High conviction that a particular set of means can be adapted to produce an asymmetric result — simple rules, unpredictable outcomes.
- Known End, Unknown Means: We know the desired outcome or change in behavior, but the challenge is identifying the most effective means to achieve that end — experiment and iterate
Let’s throw this into a classic 2×2:
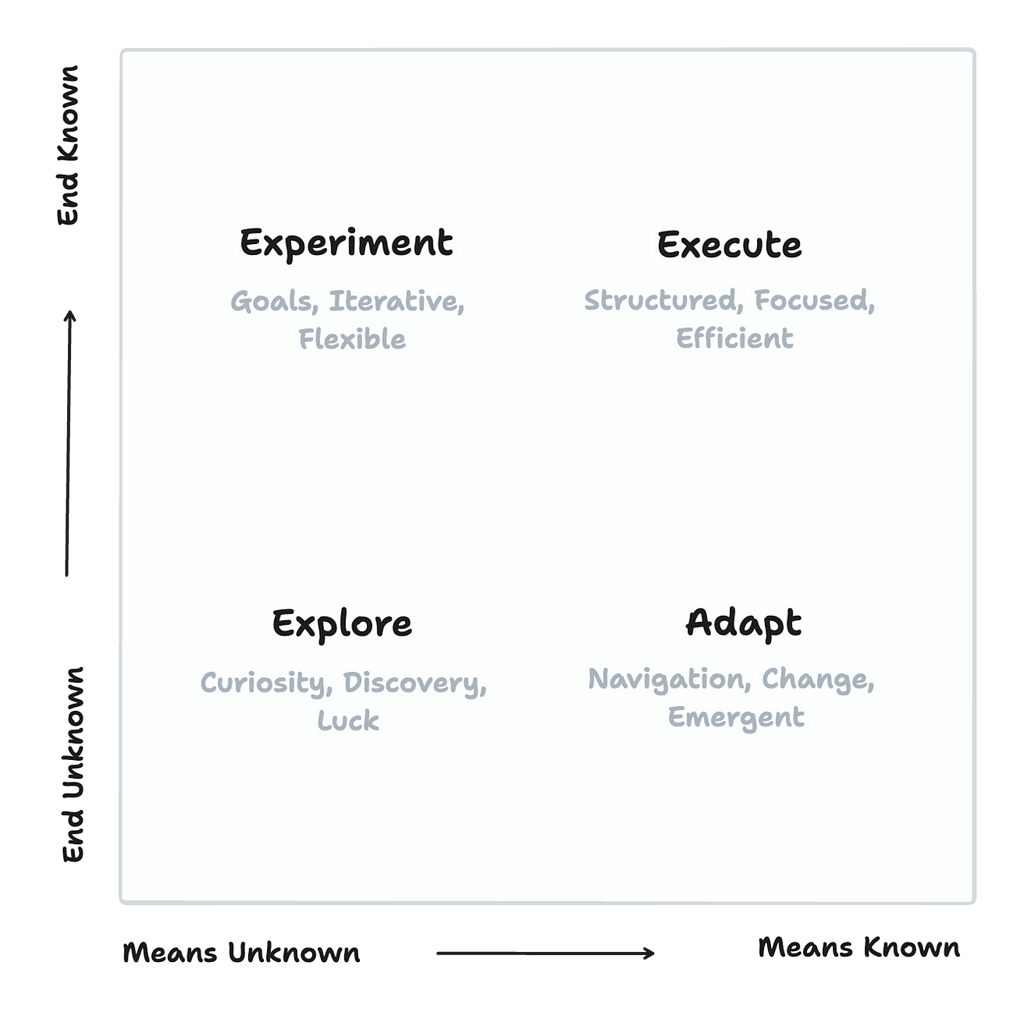
Goal-setting is an effective approach for the top two quadrants. This makes sense as they’re dependent on defining ‘known ends’, but is goal-setting as effective in the lower two quadrants?
We already gave a few examples of our ‘Explore’ quadrant here — more interactions increase the likelihood of happy accidents that come from our ‘Surface Area of Luck’ — whereby action over inaction produces some unexpected opportunity.
“You can increase your surface area for good luck by taking action. The forager who explores widely will find lots of useless terrain, but is also more likely to stumble across a bountiful berry patch than the person who stays home. Similarly, the person who works hard, pursues opportunity, and tries more things is more likely to stumble across a lucky break than the person who waits.”
— James Clear, best-selling author of Atomic Habits
Decision making in this context optimizes for action over inaction and serendipity. Given two similar choices, it asks which one produces a broader ‘luck surface area’.
That gives us:
- Known End, Known Means → Execute → Plans & Targets: Decision making is proactive
- Known End, Unknown Means → Experiment → Goal-setting: Decision making is reactive
- Unknown End, Unknown Means → Explore → ‘Luck Surface Area’: Decision Making is chaotic and random
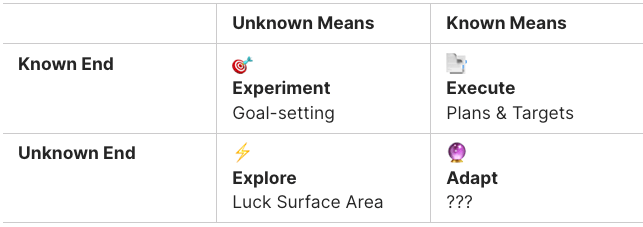
But what about ‘Unknown End, Known Means’? Decision making in this context is inherently emergent — more akin to sensemaking than optimization.
In this post, we’ll cover three perspectives on the challenges of goal-setting in radically uncertain environments.
A study done by Saras D. Sarasvathy at UVA titled, ‘What makes entrepreneurs entrepreneurial?’ explored the decision making of founders and proposes an interesting distinction between inherently exploratory (divergent) activities and ‘exploit’ (convergent) activities.
She explains this distinction as ‘Causal Rationality’ and ‘Effectual Reasoning’
“Causal rationality begins with a pre-determined goal and a given set of means and seeks to identify the optimal — fastest, cheapest, most efficient, etc. — alternative to achieve the given goal. A more interesting variation of causal reasoning involves the creation of additional alternatives to achieve the given goal. This form of creative causal reasoning is often used in strategic thinking.
Effectual reasoning, however, does not begin with a specific goal. Instead, it begins with a given set of means and allows goals to emerge contingently over time from the varied imagination and diverse aspirations of the founders and the people they interact with. While causal thinkers are like great generals seeking to conquer fertile lands, effectual thinkers are like explorers setting out on voyages into uncharted waters.”
— ‘What makes entrepreneurs entrepreneurial?’, Saras D. Sarasvathy
The argument here is that goals may be less effective in scenarios that call for effectual reasoning over causal reasoning (e.g. the ‘unknown end, known means’ quadrant).
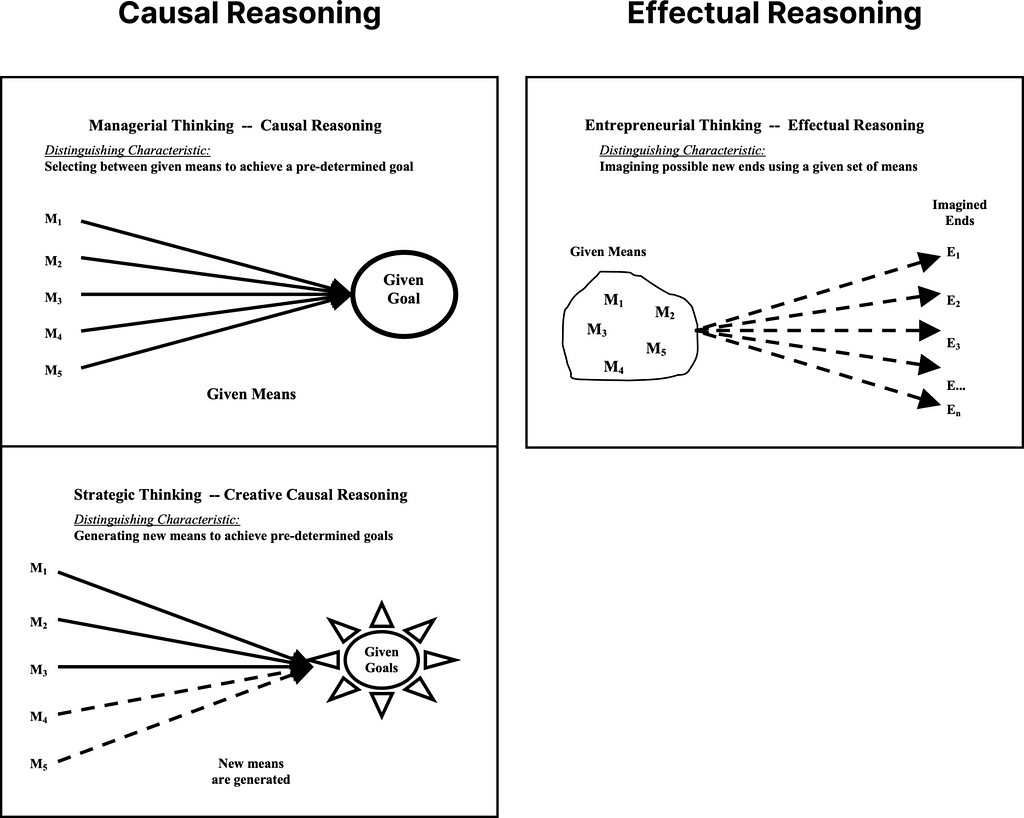
The reality is that nothing emergent and explorative tracks progress towards a target (e.g. there’s no definition of ‘on track’ or ‘off track’ when there’s no known end), so we may need different ‘effectual’ techniques in this environment — techniques that don’t define success as an end, but measure the cohesion of means that have the potential to produce desired results.
The natural tendency is to want defined signals here — like a clear north star as a reference for progress, but in reality, we need something more like ‘scaffolding’ that guides decision making.
A startup in pursuit of product-market fit (PMF) is a compelling example of this ‘known means, unknown end’ quadrant because:
- Competition is relative (goals tend to be absolute)
- Adaptability matters more than optimization (survival > best)
To navigate decision making in this problem space, founders start and adapt with known means toward an unknown end. As Sarasvathy observed, these means are often their specific traits and abilities, unique expertise, their own network/distribution, and conviction in a narrative.
“Using these means, the entrepreneurs begin to imagine and implement possible effects that can be created with them. Most often, they start very small with the means that are closest at hand, and move almost directly into action without elaborate planning.
Unlike causal reasoning that comes to life through careful planning and subsequent execution, effectual reasoning lives and breathes execution. Plans are made and unmade and revised and recast through action and interaction with others on a daily basis. Yet at any given moment, there is always a meaningful picture that keeps the team together, a compelling story that brings in more stakeholders and a continuing journey that maps out uncharted territories.
Through their actions, the effectual entrepreneurs’ set of means and consequently the set of possible effects change and get reconfigured. Eventually, certain of the emerging effects coalesce into clearly achievable and desirable goals — landmarks that point to a discernible path beginning to emerge in the wilderness.”
— ‘What makes entrepreneurs entrepreneurial?’, Saras D. Sarasvathy
2. The explore-exploit dilemma, is something missing?
The argument: The explore-exploit dilemma is often the framing for how goals support tradeoff decisions, but does this limit the learning/curiosity needed for this ‘adaptive’ quadrant?
The exploration-exploitation dilemma occurs in many of our own conscious and subconscious decision making functions — it’s also a widely referenced model for strategic decision making
We constantly balance the reward of known/unknown means and ends — but is this an ‘optimization’ problem?
We often treat decisions around goals like the multi-armed bandit problem — allocating set resources between competing initiatives to maximize expected gain and as time passes, the value of those choices becomes better understood and we weigh alternatives.
But is this effective when we’re doing more sensemaking than optimizing?
Studies on the explore-exploit dilemma have tried to define what ‘explore’ and ‘exploit’ really mean and articulate the pitfalls on either side of the equation.
In the widely cited paper ‘The Interplay Between Exploration and Exploitation’, Gupta, Smith, & Shalley summarize different definitions of ‘explore’ and ‘exploit’. Looking at our 2×2 from earlier in this post, we could argue that the top half aligns with ‘exploit’, and the bottom half aligns with ‘explore’.
Gupta, Smith, & Shalley frame the downsides on either side of the spectrum in a succinct and compelling way:
“Because of the broad dispersion in the range of possible outcomes, an exploration often leads to failure, which in turn promotes the search for even newer ideas and thus more exploration, thereby creating a ‘failure trap.’ In contrast, exploitation often leads to early success, which in turn reinforces further exploitation along the same trajectory, thereby creating a ‘success trap.’
In short, exploration often leads to more exploration and exploitation to more exploitation.”
Our fixation on pre-determined desired outcomes will likely lead us to predictable results (complacency in the success trap). Conversely, endless exploration has a hard time justifying investment when value is delayed and failure is the rule, not the exception — survivorship bias tends to have an impact here.
In entrepreneurial ventures, things like conviction help fill this gap — but we have a hard time finding a proxy for ‘effectual reasoning’ in mature organizations. The consequence of this is likely a decreased capacity for making ambitious bets.
“Objectives are well and good when they are sufficiently modest, but things get a lot more complicated when they’re more ambitious. In fact, objectives actually become obstacles towards more exciting achievements, like those involving discovery, creativity, invention, or innovation — or even achieving true happiness. In other words (and here is the paradox), the greatest achievements become less likely when they are made objectives.
Not only that, but this paradox leads to a very strange conclusion — if the paradox is really true then the best way to achieve greatness, the truest path to “blue sky” discovery or to fulfill boundless ambition, is to have no objective at all.”
— Kenneth O. Stanley, Why Greatness Cannot Be Planned: The Myth of the Objective
It’s easy to say that comfortability with uncertainty (unknown ends) presents asymmetric opportunities, but if goals are less effective, what’s the playbook for this adaptive environment?
The explore-exploit dilemma relies on a ‘reward’ as a primary driver for decision making, but a recent paper out of Carnegie Melon called ‘Embracing curiosity eliminates the exploration-exploitation dilemma’ proposes a focus on learning and ‘curiosity’.
The argument is that introducing information as a reward, or ‘learning for the sake of learning’ can outperform models that try to optimize the multi-armed bandit problem.
My background is in design and product management, not machine learning, but the compelling arguments for me were:
- There is value in learning and curiosity with no defined end
- There is a relationship between ‘explore’ and ‘exploit’ activities — though it’s inherently a tradeoff, each reinforces the other.
“Let’s consider colloquially how science and engineering can interact. Science is sometimes seen as an open-ended inquiry, whose goal is truth but whose practice is driven by learning progress, and engineering often seen as a specific target driven enterprise.
They each have their own pursuits, in other words, but they also learn from each other often in alternating iterations. Their different objectives is what makes them such good long-term collaborators.”
— Erik J Peterson and Timothy D Verstynena, Embracing curiosity eliminates the exploration-exploitation dilemma
Maybe the question here is ‘What exactly is learning progress?’ and not ‘Are we exploiting the right means to reach a desired end?’, but progress towards learning for the sake of learning that ultimately reinforces exploitive strategic decisions.
3. Goal-induced Blindness
The argument: We tend to ignore the downsides of goal-setting and in our relentless pursuite of a well-defined, measured destination, we may be subject to ‘mass irrationality’.
1996 was the highest death toll in Mount Everest’s recorded history. The stories from that year are often cited in explanations of goal-induced blindness.
The tragedies from that year were unexplainable — experienced mountain climbers ignored clear evidence and even pre-defined rules in their pursuit of the summit. In the most famous disaster that year, witnesses who saw climbers continue the ascent (that ended in the death of 8 climbers including one of their guides), were baffled to watch them seemingly disregard overwhelming evidence that their lives would be in danger if they didn’t turn around.
What happened?
After the disaster, Chris Kayes, an expert in management and organizational behavior, proposed they may have been ‘lured into destruction by their passion for goals.’
In his book, Destructive Goal Pursuit: The Mt. Everest Disaster, Kayes coined the term goalodicy — defined as, ‘the obsessive pursuit of goals to the point of self-destruction.’
Kayes’ argument is not that goals are inappropriate or always detrimental, but that leaders need to be thoughtful about when they are effective. In environments that revere goal achievement, research suggests that goals often lead to critical failures — disasters such as the 1996 Everest climb, the Columbia disaster, and organizational collapses like Enron.
Kayes’ alternative focuses on complimenting goal-setting with mechanisms for team learning and adaptation over goal achievement — which he summarizes in these points:
- Setting and pursuing high and difficult goals often drive failure, not just success
- Learning and adaptation, not vision alone, lie at the heart of leadership
- Effective teamwork and learning, not simply goal-setting, leads to success in the face of novel situations.
In summary, we tend to ignore how often audacious goals lead to unintended consequences and failure, learning should be the focus over achievement, and we need different approaches when we’re facing novel situations.
4. Escalation of commitment
The argument: Goals can trigger an escalation of commitment (commitment bias) — where we can over-invest in early wins, ignore challenges that may change our objectives, and take deterministic approaches to achieve desired outcomes.
There are powerful cognitive mechanisms involved that transfer from individuals to groups when goals are involved — one of which is the subject of Annie Duke’s book, Quit, where she makes the case for a disclaimer:
Clearly defined goals should come with a warning: Danger: You May Experience Escalation of Commitment
— Annie Duke, Quit: The Power of Knowing When to Walk Away
It’s common to reference the sunk-cost fallacy when it comes to project investments or initiatives, yet we tend to disregard our tendencies to overcommit when it comes to goals.
OKRs are typically crafted at a single point in time, where the variables in the environment are often implicit. Meaning the OKRs assume that these variables will stay the same. In economics, this is explicitly stated as ceteris paribus or ‘all other things being equal’ to represent the constant change in other variables (known or unknown).
When this is implicit, what we’re saying is ‘given this is true’, but as variables change, we tend to keep the assumptions built on them. Some of these variables are salient — of course, we’re going to challenge our existing goals and strategy with the introduction of generative AI — but others are much more subtle.
The argument here is not against goal-setting — it’s advocating for goals designed to be resilient to inevitable change. As economist John Kay argues, sometimes the best way to achieve objectives is to do so indirectly.
Kay describes this concept as obliquity. The premise is that objectives are complex and rarely well-defined. Complex solutions typically emerge through trial and error — not by evaluating options and selecting the ‘right’ solutions. As we’re exploring solutions, we tend to learn more about the goals we’re trying to achieve.
Therefore, the deterministic approach to goal-setting can lead us down a predictable path — a path that tends to feed our escalation of commitment, even if it clearly isn’t working.
Why? Because a deterministic approach isn’t designed to reward the identification of what isn’t working — it rewards finding the right solution — which incentivizes doubling down on early, small wins that snowball into large mediocre initiatives.
OKRs can easily provide a facade of an outcome-driven, experimental environment when in reality, we just find something (anything) that can present as ‘on track’ towards a goal and stick with it.
John Kay’s response to this problem is pluralism over monism — meaning there are many different ‘right’ solutions that exist, not a single optimized solution. This means our choices should more often than not be between multiple good options, not a hunt for the ‘right’ choice.
Therefore, Kay argues, high-level goals should be approached indirectly through the means — or as Charles E. Lindblom describes as ‘the science of muddling through’:
“Initially building out from the current situation, step-by-step and by small degrees.”
— Charles E. Lindblom
This is a challenge-based mindset (what would change our mind about this?) vs. a validation mindset (what is going to confirm we’re right about this?). This is a subtle, but powerful difference.
5. Distorted incentives and risk preferences
The argument: Goals create incentives that may drive unintended behavior. Studies and use cases show the dark side of goal-setting where huburis combined with audacious goals was a recipe for disaster, but there may be another side to that coin — goals may also unintentionally create incentives that reduce risk appetite and favor complacency.
The fact that audacious goals can promote unethical behavior and lead to disastrous outcomes shouldn’t come as a surprise.
If we assume positive intent in all scenarios, we still wouldn’t argue that the ends justify the means.
If we imagine that Sam Bankman Fried, the founder of FTX currently facing a possible life sentence for financial fraud, was truly acting in accordance with his audacious goals aligned to ‘effective altruism’, we wouldn’t justify the alleged misappropriation of customer funds to do so — even if it had gone unnoticed.
This is a concept that famed management researchers and professors Ordóñez, Schweitzer, Galinsky, and Bazerman describe as ‘Goals Gone Wild: The Systematic Side Effects of Over-Prescribing Goal Setting’.
They have a provocative opening to their paper:
“Goal setting is one of the most replicated and influential paradigms in the management literature. Hundreds of studies conducted in numerous countries and contexts have consistently demonstrated that setting specific, challenging goals can powerfully drive behavior and boost performance.
Advocates of goal setting have had a substantial impact on research, management education, and management practice. In this article, we argue that the beneficial effects of goal setting have been overstated and that systematic harm caused by goal setting has been largely ignored”
— Ordóñez, Schweitzer, Galinsky, and Bazerman, Goals Gone Wild: The Systematic Side Effects of Over-Prescribing Goal Setting
The paper details the areas of vulnerability with goal-setting frameworks. This is different than ‘pitfalls’ that suggest a framework is implemented incorrectly — meaning it’s important to point out that we can assume a framework like OKRs is done correctly and still observe these possible side effects.
Granted, in my experience, I think something like OKRs has likely had more real-world adaptation to these issues than goal-setting has in the past (this paper was published in 2009), but it’s interesting to dig into the criticisms made here:
- When goals are too specific and narrow: The argument is that narrow goals can cause systemic inattentional blindness — the concept that we’re unaware of the power of our focus. Studies show that when we’re faced with specific tasks, we often ignore obvious stimuli as shown in the classic ‘awareness test’ of a team passing a basketball.
- Too many goals: When multiple goals are present, there may be unintended consequences as we tend to only focus on one single goal. For example, in a multi-goal situation, we tend to sacrifice ‘quality’ goals when ‘quantitative’ goals are present.
- Inappropriate time horizons: “Goals that emphasize immediate performance prompt managers to engage in myopic, short-term behavior that harms the organization in the long run”. People and teams tend to see goals as a ceiling of performance rather than a floor.
- Level of challenge and risk-taking: “Goal-setting distorts risk preferences … People motivated by specific, challenging goals adopt riskier strategies and choose riskier gambles than do those with less challenging or vague goals.”
- Learning and cooperation: “Locke and Latham recommend that ‘learning goals’ should be used in complex situations rather than ‘performance goals.’ In practice, however, managers may have trouble determining when a task is complex enough to warrant a learning, rather than a performance goal. In many changing business environments, perhaps learning goals should be the norm.”
- Harming intrinsic motivation: “Although people recognize the importance of intrinsic rewards in motivating themselves, people exaggerate the importance of extrinsic rewards in motivating others. In short, managers may think that others need to be motivated by specific, challenging goals far more often than they actually do. By setting goals, managers may create a hedonic treadmill in which employees are motivated by external means (goals, rewards, etc.) and not by the intrinsic value of the job itself.”
OKRs, as an evolution and adaptation of ‘Management by Objectives’ (MBO), take many of these side-effects into account. The framework makes recommendations to reduce the number of goals (recommending groups stick to a 3–5 limit), break down time horizons with check-ins, and take a fail-fast learning approach to achieve goals, but even when implemented ‘by the book’, the psychological effects of goal-setting can have unintended side-effects.
As Ordóñez, Schweitzer, Galinsky, and Bazerman suggest, OKRs should be handled as a potent prescription — it’s an incredibly powerful method for influencing motivation, cooperation, and ultimately outcomes, but it should be prescribed with caution and consideration of the negative side effects.
“There are many ways in which goals go wild: they can narrow focus, motivate risktaking, lure people into unethical behavior, inhibit learning, increase competition, and decrease intrinsic motivation. At the same time, goals can inspire employees and improve performance. How, then, should we prescribe the use of goal setting? Which systematic side effects of goal setting should we most closely monitor, and how can we minimize the side effects?
Just as doctors prescribe drugs selectively, mindful of interactions and adverse reactions, so too should managers carefully prescribe goals. To do so, managers must consider — and scholars must study — the complex interplay between goal setting and organizational contexts, as well as the need for safeguards and monitoring.”
— Ordóñez, Schweitzer, Galinsky, and Bazerman, Goals Gone Wild: The Systematic Side Effects of Over-Prescribing Goal Setting
As I’ve said throughout these posts, I’ve seen first-hand how powerful OKRs are; both as a practitioner and while building OKR management experiences for Atlassian’s largest customers. I believe OKRs have a place in every organization, but I do believe they are overprescribed into contexts where they may not be as effective — for example, when used as a substitute for strategy or in problem spaces where the focus should be on the means, not the ends.
As with anything that builds a strong following and becomes unquestioned standard practice, it’s helpful to humble our perspectives and look at the counterarguments.
In the case of OKRs, there are decades of research on goal-setting in management practices. I’ve found healthy new perspectives in exploring these arguments, and I hope you did as well.
This post was originally published on 🔮 The Uncertainty Project — a resource on tools and techniques for strategic decision making and navigating uncertainty.
Are OKRs improving or inhibiting decision-making? was originally published in UX Collective on Medium, where people are continuing the conversation by highlighting and responding to this story.
Leave a Reply