The art of deception explained & why some “health” foods might not be as healthy as you thought.
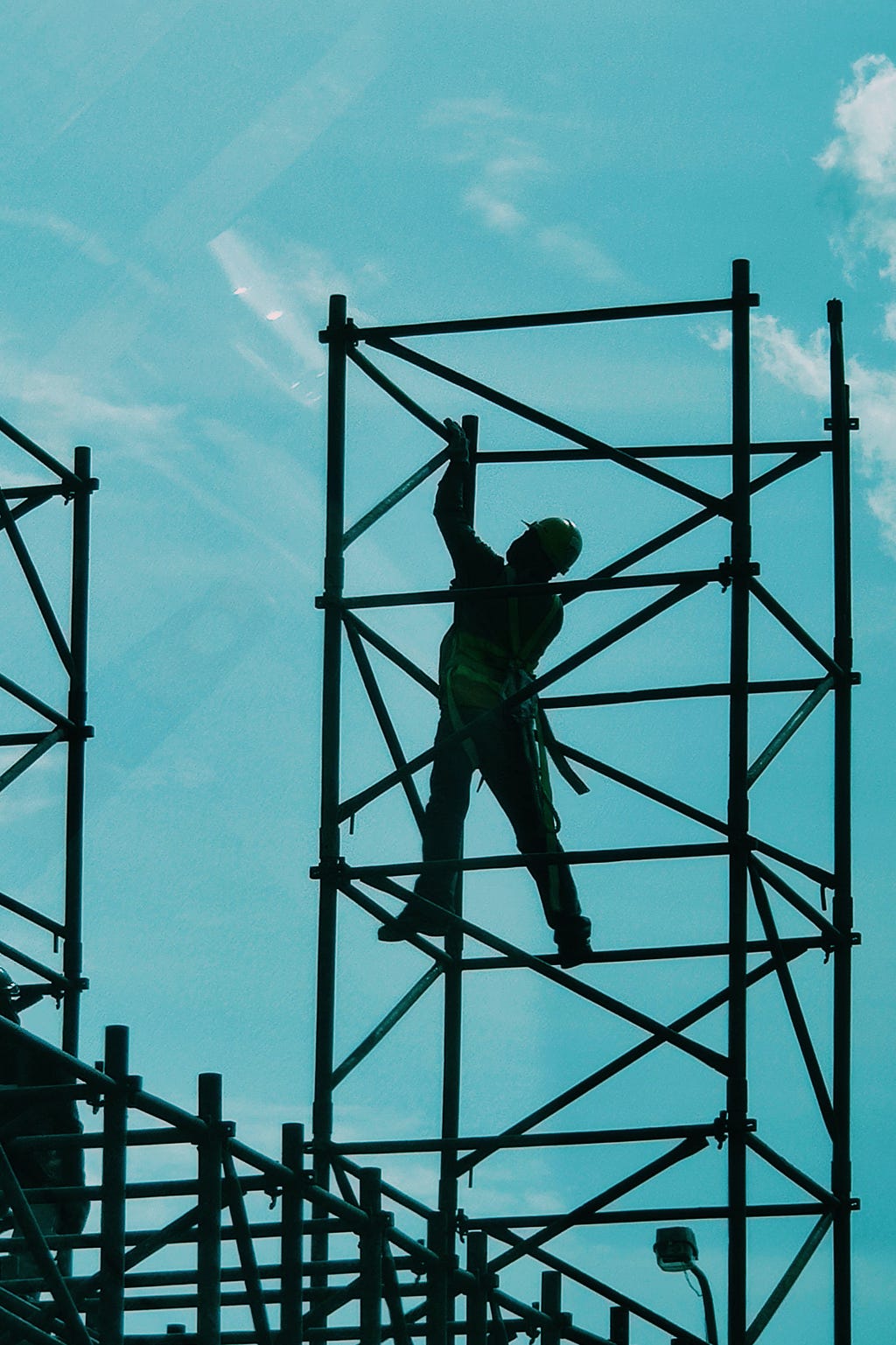
I’m writing this at home in my fourth-story apartment. When I do, I’m trusting that my building isn’t going to collapse on me.
I’m putting my faith in the rigor of my building’s construction.
The same is true when last week I rode an elevator to the 57th floor of a skyscraper downtown. Or this past weekend when I went underground and took the subway to see a friend. And chances are pretty good that you trust your life to rigorous construction as well.
When we do that, what are we trusting exactly? Well, we trust that the architects and engineers have designed the project in such a way so that it’s stable and enduring. In other words, we trust that there is rigor in design.
But superintendents, field engineers, labourers, and many other folks on construction projects have to create the actual, physical thing based on the designs. So, we also trust that those individuals are translating the designs accurately, with care and consideration — that they’ll ask for clarification to the architects and engineers on anything ambiguous while erring on the side of caution and safety. When we do that, we’re trusting that there is rigor in interpretation.
It’s a subtle distinction, but reasoning in this way can lead us to an important insight about research rigor.
Rigor in design vs. rigor in interpretation
When I searched “UX research rigor” on Medium to prep for this story, I found stories about rigor in creating survey instruments and rigor in planning and executing user interviews. These stories are about rigor in (research) design: The careful selection of the appropriate method(s) for a given set of research questions, and then implementing that method appropriately and effectively.
This story is not about that — not because rigor in design isn’t important (it absolutely is) but because I want to draw attention to something slightly different.
A rigorous set of specs for a construction project is crucial, but so is the proper interpretation of those specs. Similarly, it’s not enough for researchers just to execute a well-designed study.
Our job isn’t just to own how research is done, but also how research is understood and used.
Researchers are evaluated on their impact. But research impact is seen in the decisions that stakeholders take based on research, and those stakeholders make their decisions using the pictures in their heads — their own interpretation and understanding of what you found.
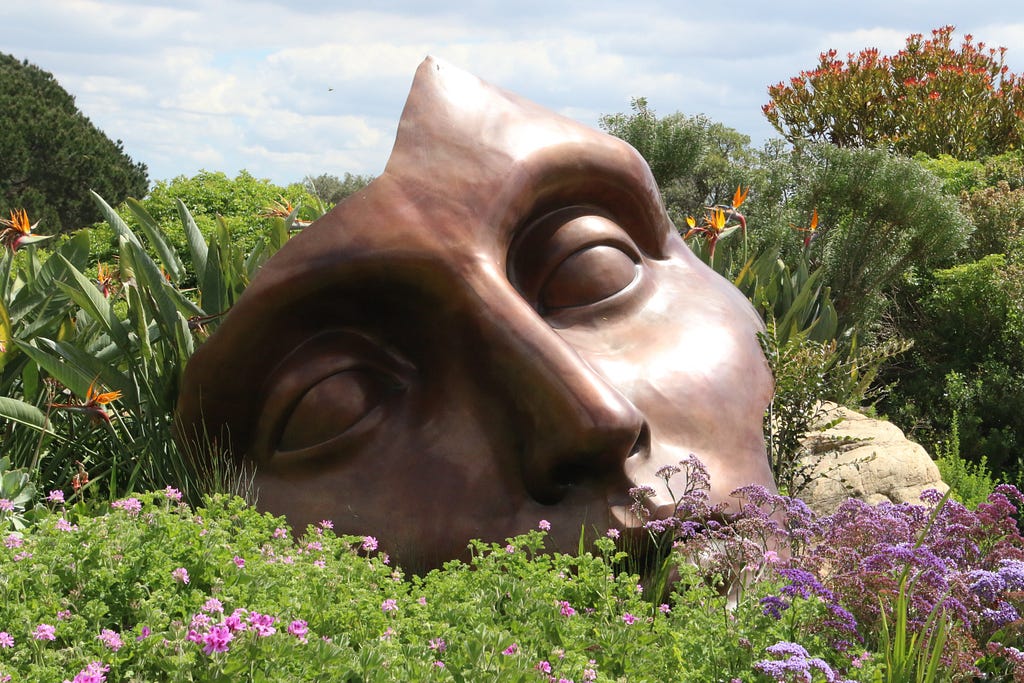
We can’t control what stakeholders think, but we can influence it: We can educate them on accepted standards, on why we can have high confidence on certain findings and lower confidence on others. We can steer our stakeholders when they veer off-track, and we can be a navigator and a translator for our team on not just findings, but specific interpretations and implications of findings.
Practically, there are a couple of specific pieces of evidence I look for to see if UX researchers (and People Who Do Research) are interpreting findings rigorously:
- Conservatively and cautiously drawing conclusions from findings
- Being honest, clear, and upfront about limitations of a specific research design/study, and what those limitations mean for what we should take away
- Incorporating reflexivity and positionality into the conduct of user interviews and when analyzing the resulting data
- Considering alternative explanations for observed results
- Challenging their own interpretation — constantly
(This list certainly isn’t comprehensive, but it’s a solid starting point).
A real-world example
Here’s an example of when I recently struggled with rigor in interpretation. (The numbers here are fake but all the other details actually happened — it’s the event that inspired this story).
Our team was fielding an A/B/C test of a new feature. Users were randomly assigned to one of three experimental groups:
- Control group (who saw the product as it exists today)
- Treatment A (who saw one version of the new feature)
- Treatment B (who saw a different version of the new feature)
The results looked like this:
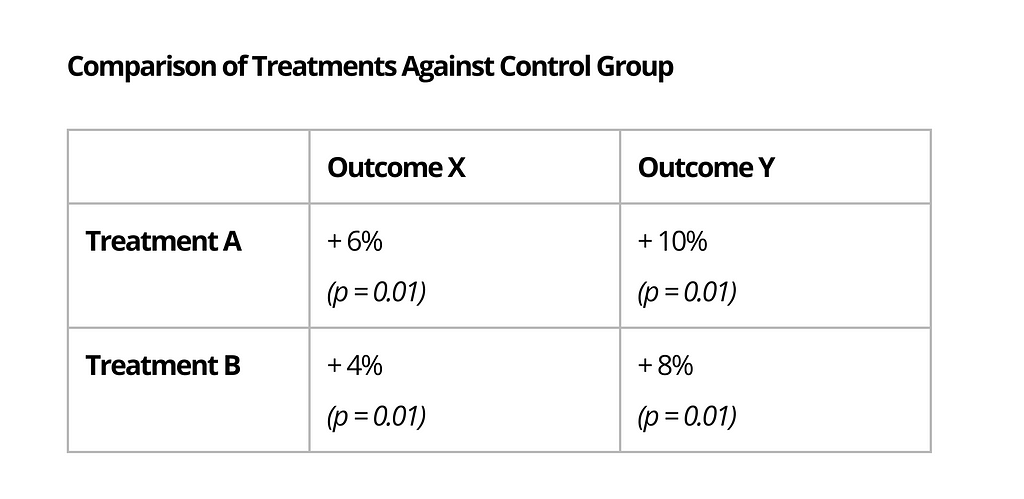
Based on this table, our product team concluded that Treatment A outperformed Treatment B.
Looking at those results, would you agree?
What if I told you that the comparison of the two treatment groups against each other looked like this:
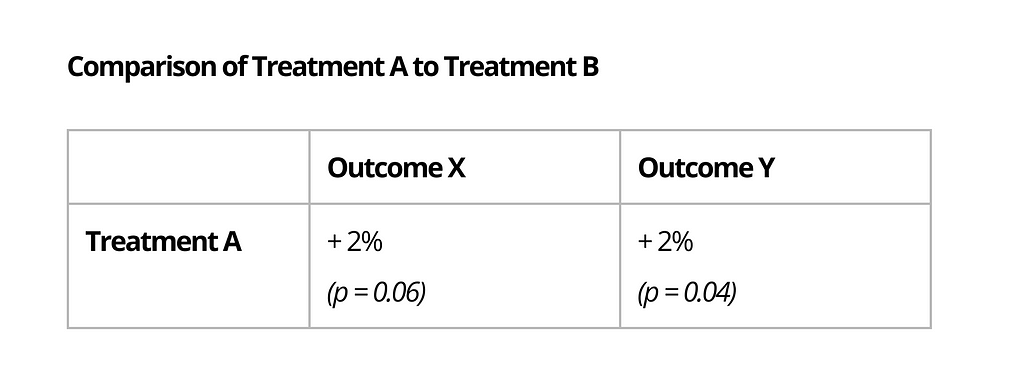
What if I also told you that the tool we are using to conduct the experiment and to analyze this data is using t-tests for these tests of statistical significance — an inappropriate test when there are more than two groups?
***
Maybe this feels a little “mountain out of a mole hill” to you at this point, and I don’t necessarily disagree. But when people are moving fast and breaking things, I have no qualms being the party-pooper-cautious-voice-of-reason.
Sometimes, that’s what the team needs. (I work in gaming, but let’s say you work in health and your “Outcome X” is something like, say, depressive episodes. Or subjective pain. Or mortality. I’d want you to be a party pooper in that case!)
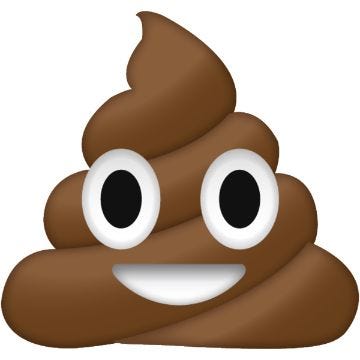
It was also important to me to set a precedence for rigor when interpreting experimental results. I won’t be so close to the results of every A/B test our team runs, and certainly not every A/B test run by our PMs throughout their careers. The mole hill won’t always be a mole hill. The products we work on structure and affect so much of our lives — how we work, how we shop, how we date, how we interact with others — that being rigorous in how we interpret results and make decisions based on those interpretations is one very real, tangible way that UX researchers can make a difference on people and in the world.
(For what it’s worth, my answer to Product here was something like, “If you put a gun to my head, I’d pick A. But I genuinely don’t think it’s as clear cut as it might seem at first glance because the estimate for the difference between the two treatments is small and less precise, and the test the tool is using will underestimate the error and overstate the confidence. This result gives us a hint, but we don’t have to discard B if there are other factors in its favour, like cost or design debt.”)
Three rules of thumb to maintain rigor in interpretation
I attribute my own sense and ideas about rigor in interpretation to my academic training. And if I were to distill those ideas down into three rules of thumb, it’d be these:
- Err on the side of safety
Results are rarely clear-cut. Our quantitative data are often messy, with multiple outcomes and metrics that, ideally, would point in one direction but don’t always. Our qualitative data can be even worse — turns out humans are complicated.
In these cases, hold yourself and your team to a high standard for evidence. Be honest about the messiness and the level of confidence we can have.
What “safety” means is of course relative, and being safe does not mean decision paralysis or not having a voice. Ask yourself what decisions your team will take based on this research. Are you facing a one-way or two-way door? How would a bad decision affect your team and your users?
2. Advocate not just for findings, but specific interpretations of findings
Being rigorous does not mean avoid offering a point of view. It’s actually the exact opposite. If researchers only stick to statements like “the results show X,” we leave the meaning of X up to others. Doing so is a surrender of power, and yet it doesn’t absolve us of responsibility.
Finish that previous statement with a “and X means Y,” and remind your team about Y constantly — in syncs, in 1-on-1s, in future shareouts and deliverables.
Remember, we can’t control the picture in their heads, but we can and should try our best to align those pictures with our own.
3. Be open to being wrong
Being an advocate is also not the same thing as being dogmatic. Ambiguity is where a lot of research lives, and researchers are also human.
Many people — particularly those who are junior — fear being wrong. This is true not just for UXRs but across pretty much every role that I’ve interacted with. I suspect it’s what makes receiving feedback so hard for some.
The truth is, to be human is to be wrong at least some of the time. At the end of the day what truly matters is making the best possible decision for your team and for your users. You can’t do that if you think you’re always right.
***
Rigor in how we design research alone isn’t enough — we need to be rigorous in how we interpret research findings as well. Doing both is part of the job, and it’s how we create good research.
What does “rigorous” research really mean? was originally published in UX Collective on Medium, where people are continuing the conversation by highlighting and responding to this story.
Leave a Reply