Supercharging already unequal and discriminatory systems through AI.
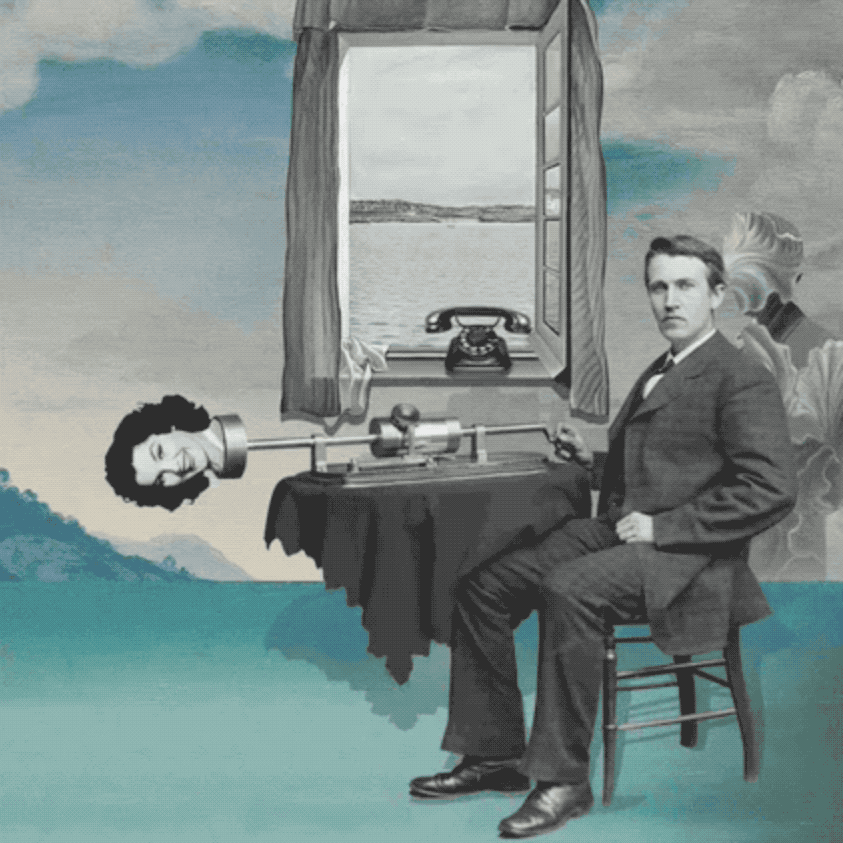
Today, humans interact much more with AI models rather than other humans. Whether for entertainment on Youtube and Netflix, inspiration on Instagram and TikTok or for finding a partner on Tinder and Hinge, an AI model decides which humans and content we should interact with. AI already controls our lives in the background without us even realizing it.
We outsourced our decision-making to algorithms, and now they decide which humans get housing or healthcare insurance, who gets hired or admitted into colleges, our credit scores, etc. The hope was that by removing humans from the equation, the machines would be able to make unbiased and fair decisions; however, this is not the case. The data used to train these models comes from a fundamentally biased society; it is impossible to eliminate them entirely. The AI is just reflecting them back to us.
https://medium.com/media/b7e2a24f5613a3d48b9b6828eb504122/href
Algorithmic racism
Racist algorithms are sending people to jail
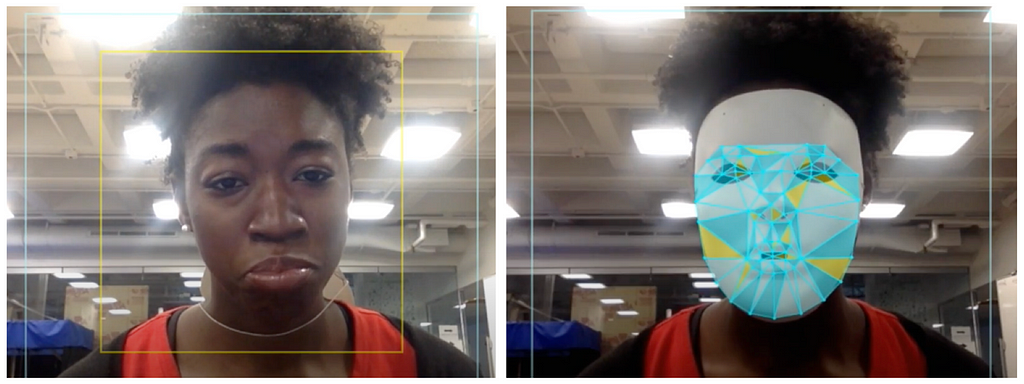
In 2015, Joy Buolamwini at the Media Lab discovered that all the face recognition algorithms at the time from IBM, Microsoft, etc., were unable to detect her face, being a darker female. However, when she wore a white face mask, they all started to see her. She noticed a 35% error difference when it came to detecting darker female faces as opposed to white males. There are many other examples from our past:
- Google’s image tagging algorithm from 2015 labeled a pair of black friends “gorillas.”
- Flickr’s system made the same mistake and tagged a black man with “animal” and “ape.”
- Nikon’s cameras, designed to detect whether someone blinked continually, told an Asian user that her eyes were closed.
- HP’s webcams from 2009 easily tracked a white face but couldn’t see a black one.
https://medium.com/media/87e176cc89ad24095db234da1866c6cc/href
“We will not solve the problems of the present with the tools of the past. The past is a very racist place. And we only have data from the past to train Artificial Intelligence.”
— Trevor Paglen, artist and critical geographer
Biased facial recognition algorithms are even wrongfully arresting black men and sending them to jail. Below are two examples of such racist decision-making tools used by law enforcers in the US:
- COMPAS: A recidivism prediction software used by judges and parole officers in the US to determine how risky criminal defendants are; this then influences their sentencing. The algorithm unfairly classifies Black and Hispanic individuals as riskier than others.
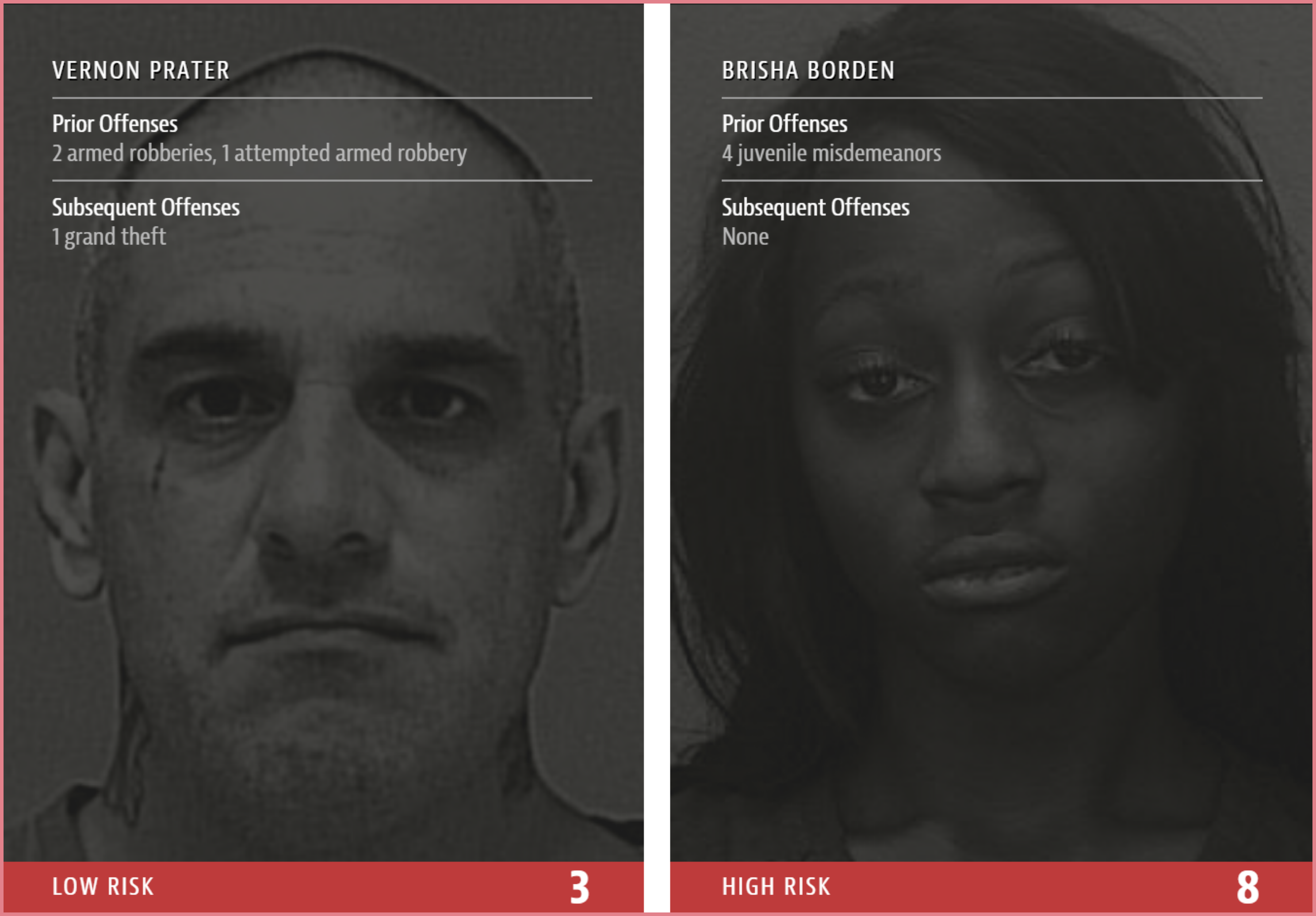
- PredPol: Predictive Policing is a “minority report” style crime prediction software used by the police to determine which neighborhoods should have more patrolling than others. Black communities always end up being more patrolled and harassed because of this biased algorithm.
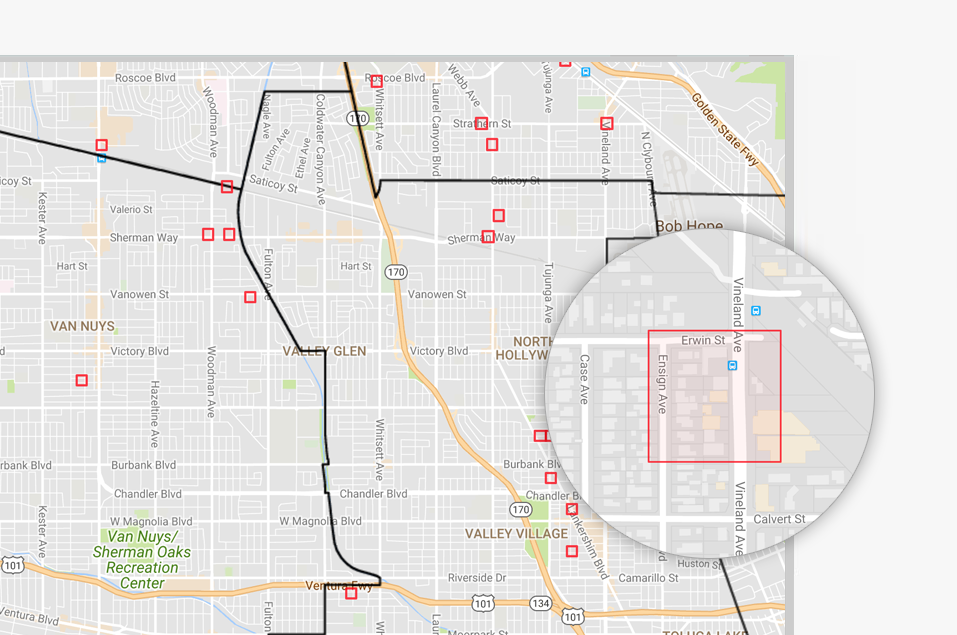
Black men are six times more likely to be incarcerated by police than white men and 21 times more likely to be killed by them.
— Cathy O Neil, Weapons of Math Destruction
Saturday Night Live – SNL on Twitter: "This is a damn trap pic.twitter.com/UssHF5MtNk / Twitter"
This is a damn trap pic.twitter.com/UssHF5MtNk
Gender bias
Binary algorithms reinforce stereotypes
Sunspring was the first ever short film written by an AI. Oscar Sharp trained the model with Sci-Fi film scripts to create this. In 2021, when he tried to do something similar by training the model with the scripts of action movies, he was flabbergasted. He noticed how all the generated stories had just a solid masculine lead, while the only female character (the romantic interest) was merely addressed as “girlfriend” throughout the story.
https://medium.com/media/d3d1b7054eb64d304fcdeb5f91870f3c/href
The OpenAI researchers themselves identified the top 10 biased words the software produces when describing men and women. For men, it’s words like “large,” “mostly,” “lazy,” “fantastic” and “eccentric.” And for women, it’s “optimistic,” “bubbly,” “naughty,” “easy-going” and “petite.” The researchers wrote, “We found females were more often described using appearance-oriented words.”
Bindu Reddy 🔥❤️ on Twitter: "Machine learning models learn the biases back in data and reflect them back to usHungarians have no gendered pronouns but apparently, Google Translate has learnt all the gender stereotypes! 😱😱 pic.twitter.com/Hi0r62PMpF / Twitter"
Machine learning models learn the biases back in data and reflect them back to usHungarians have no gendered pronouns but apparently, Google Translate has learnt all the gender stereotypes! 😱😱 pic.twitter.com/Hi0r62PMpF
Transphobic algorithms
We already live in a cissexist world, but AI is making it worse.
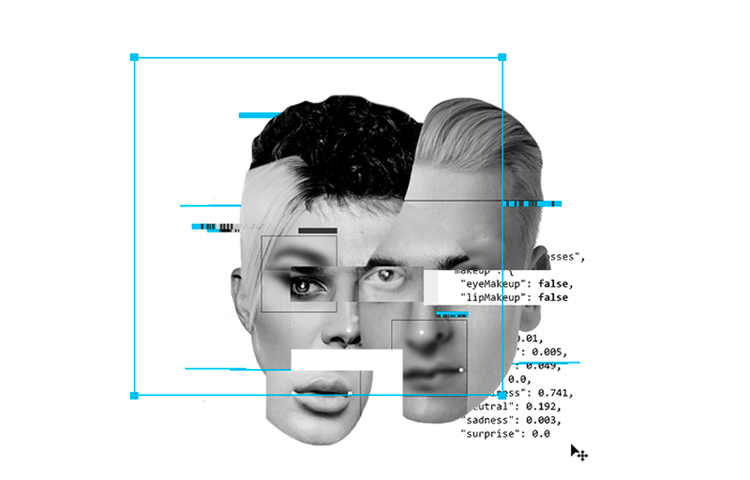
The Automatic Gender Recognition (AGR) system is the best example of transphobic algorithms. AGR is widely used for identity verification in public places like airports, malls, etc. It creates a lot of humiliation and discomfort for non-binary people when the algorithm cannot label their gender, thereby alarming and attracting more attention to them.


“Every trans person you talk to has a TSA horror story. The best you can hope for is just to be humiliated; at worst, you are going to be harassed, detained, subjected to very invasive screenings, etc. Trans people or gender non-conforming people who have a multi-racial or Middle Eastern descent are most probable to have a negative airport security incident.”
— Kelsey Campbell, Founder Gayta Science
Read more on transphobic algorithms:
- AI software defines people as male or female. That’s a problem by Rachel Metz, CNN
- A transgender AI researcher’s nightmare scenarios for facial recognition software by Khari Johnson, VentureBeat
- Counting the Countlessby Os Keyes, Real life mag
Biased hiring algorithms
If you are a white man named Jared who played high school lacrosse, you got the job!
The idea behind using AI-powered resume screening tools was inspired by blind auditions, how orchestras select musicians from behind closed curtains. This lets them look past the artist’s gender, race, reputation, etc. However, most hiring algorithms inevitably drift toward bias.
An example of this is Amazon; they have been infamous for both their hiring and firing algorithms. Hiring algorithms that prefer white males over other demographics of people and their firing algorithms that fire factory workers without clear reasoning and with little or no human oversight.
After the company trained the algorithm on 10 years of its own hiring data, the algorithm reportedly became biased against female applicants. The word “women,” like in women’s sports, would cause the algorithm to specifically rank applicants lower.
— Companies are on the hook if their hiring algorithms are biased, QZ
Who’s fighting back?
AI auditing is one of the most widely accepted techniques to mitigate bias. This framework is widely used to determine if AI models are biased, discriminatory or have other hidden prejudices. Companies like Meta and Microsoft have internal auditing teams that analyze these models, but external agencies like ORCAA, ProPublica, NIST, etc., also do it. AJL’s (Algorithmic Justice League) paper “Who audits the auditors” describes in detail what the AI auditing ecosystem looks like.
https://medium.com/media/5cb3695b1c5dc0f2a70d76ea7619381d/href
Here are some other examples of initiatives aimed at mitigating bias in AI:
- Standards for Identifying and Managing Bias in Artificial Intelligence: Guidelines from NIST designed to support the development of trustworthy and responsible AI.
- Themis: An auditing software created to engineer out bias from algorithms, revealing hidden imbalances in AI models.
- Diffusion bias explorer: A project from Sasha Luccioni from HuggingFace that reveals hidden biases within Stable Diffusion.
- The Princeton web accountability and transparency project: Bots that masquerade online as Black, Asian, poor, female, or trans and try to represent these minorities in unrepresented data sets.
- Feminist dataset: A project that includes the community in creating datasets to have a transparent approach to data collection.
- Ellpha: A project aimed at creating gender-balanced datasets that are representative of the real world.
- Queer in AI: Raising awareness of queer issues in AI/ML, fostering a community of queer researchers and scientists.
- Gayta science: Using data science techniques to capture, combine, and extract insight to give LGBTQ+ experiences a voice.
- Q: The first genderless voice assistant trained on the voices of people who identified themselves as androgynous. These voices range between 145 Hz and 175 Hz, right in a sweet spot between male and female normative vocal ranges.
https://medium.com/media/28a242b032c59780e345f07a315daf45/href
Biased algorithms are being used as a cloak for unfair practices and for adjudicating minorities. Through the above examples, the hope is that you can start questioning the decisions made by an AI. So the next time someone says that this is a fair decision made by an objective machine, it doesn’t become a conversation ender; instead, it starts a whole new discussion around bias in AI.
High-tech tools have a built-in authority and patina of objectivity that often lead us to believe that their decisions are less discriminatory than those made by humans. But bias is introduced through programming choices, data selection, and performance metrics. It redefines social work as information processing, and then replaces social workers with computers. Humans that remain become extensions of algorithms.
— Virginia Eubanks, Automating Inequality
*For further resources on this topic and others, check out this handbook on AI’s unintended consequences. This article is Chapter 2 of a four-part series that surveys the unintended consequences of AI.
Bias in AI is a mirror of our culture was originally published in UX Collective on Medium, where people are continuing the conversation by highlighting and responding to this story.
Leave a Reply