How can you design for AI in your existing journeys?
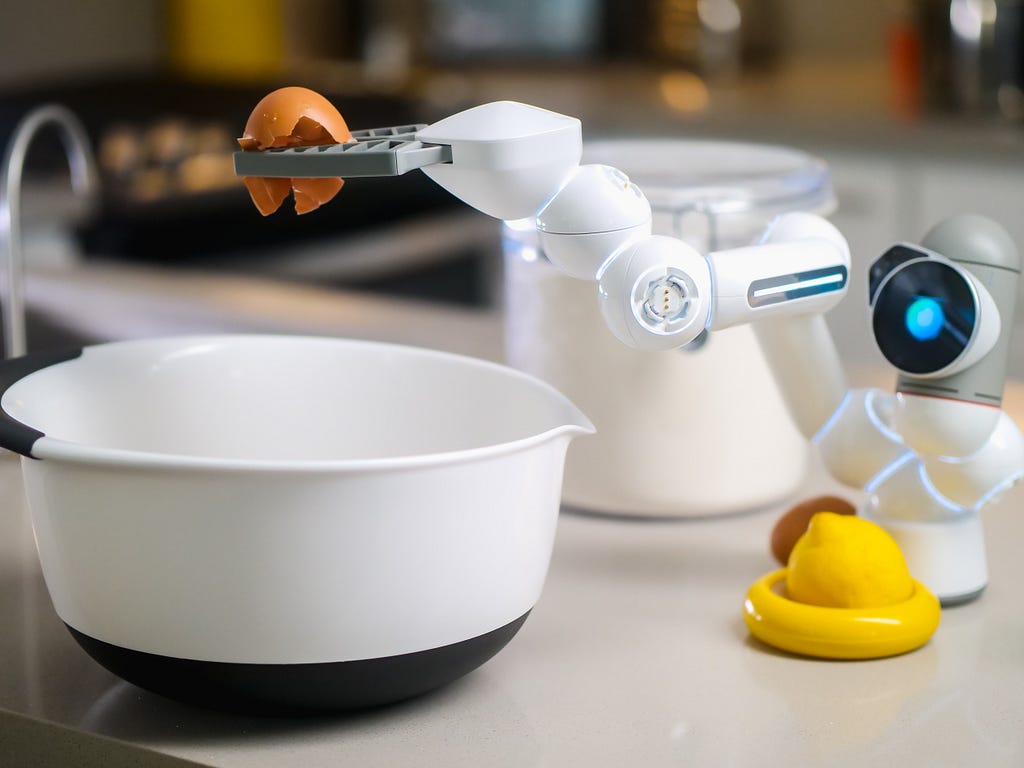
AI is here with us, and UX designers need to design for it in case they want to stay relevant in the market. But how can we AI “enable” existing products, especially in the B2B sector?
The cases of AI
Let’s provide a simple framework. Essentially, from a UX perspective, there are three cases of AI
When a UI is built on top of an AI backend
Examples of this: tools like Adobe Firefly or DALL-E, first-generation integrations like Miro AI or Notion’s AI.
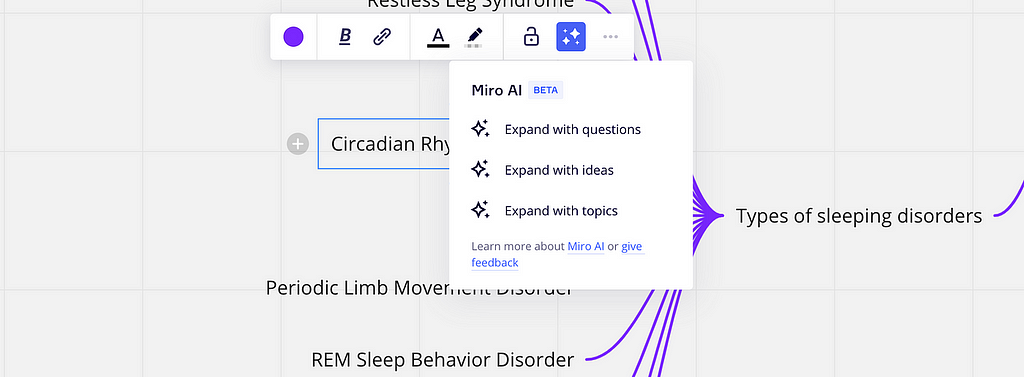
This is typical: you have a traditional system, let’s say, a form, a list of things, or some text, you put it through AI and get back the results, presenting on the UI.
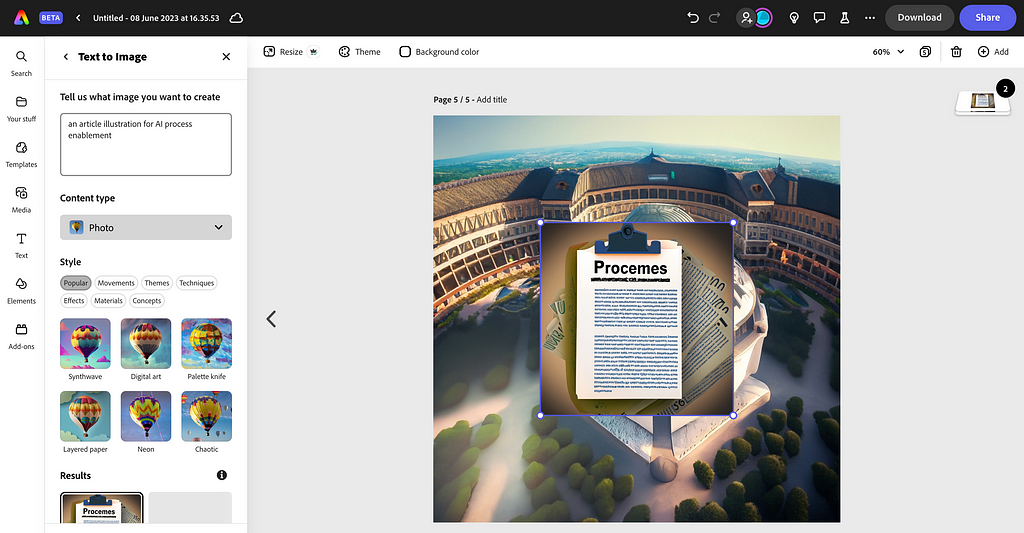
The point here is that it still feels like a traditional software mostly, with some nice autocomplete tools. UI is mostly used to translate user input into something AI can be consistently prompted with, and using that consistent prompt, we get back some results which are, again, processed into a conventional format.
The good thing about this, that it is very easy to prototype it: all it takes is a bit of clever prompt engineering.
Do it in current AI tools, record it in a video or share your ChatGPT transcript to developers and tell them that this is what you want them to build.
When AI is the UI of a traditional backend
Examples of this: all ChatGPT plugins, customer support chatbots built on AI, AI-enabled search products, Apple’s SIRI, all voice-recognition-enabled tools, Apple and Google photo search
This is usually a conversational interface: an AI acts as a “helpful assistant”, but it uses traditional computing tools, e.g. checks data in a database. AI is mostly used to translate user input into a consistent format and to translate the coming output into a human-friendly format, or categorize into one of the UI display formats (e.g. which SIRI widget to display), perhaps highlighting key information (e.g. both “Will it rain?” Or “What’s the temperature outside?” will get you back the same weather widget, but the AI might highlight different information).
It’s a bit technical, but it’s also easy to prototype. I was able to provide a AI frontend for public transportation, but I’m still waiting for my GPT-4 API key and the ability to write my own plugins 🙁
This is an example transcript on how AI can act as a waiter. It won’t take long before Wolt or Foodora or other food delivery solutions will provide an AI-enabled experience.
When AI replaces/automates steps or complete workflows
Examples of this: text generators, translators, robot psychologists (e.g. woebot), routing on Maps (yes, routing/directions are AI), image recognition software
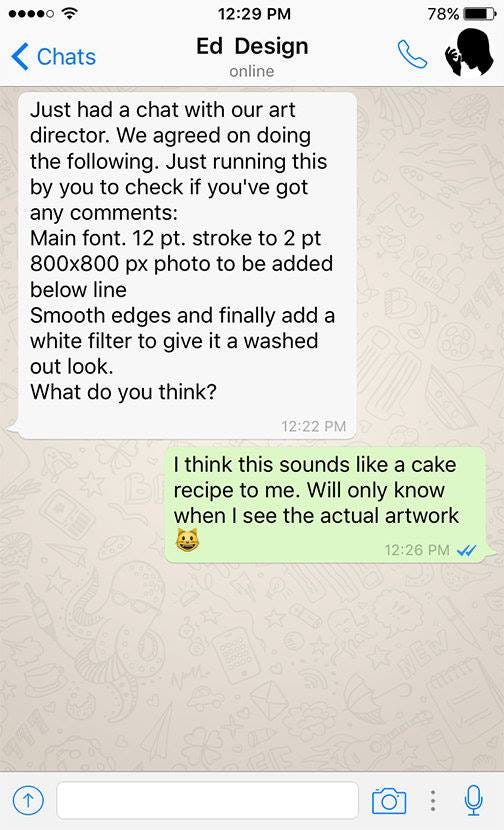
This is when you go to midjourney and talk it into designing your new logo. Essentially, you use AI like you would talk to a junior guy over Slack: you assign tasks, the AI solves it, you might ask changes, the UI does them.
The UX challenge is always to provide the correction loop so that we can either get to a solution through trial and error, refine the request, or ask changes.
Also, it is crucial to have a review stage: AI is prone to bullshitting, to the level which might feel not only surprising but perhaps even alien to all those who never consulted mid-to-low performing undergraduate assignments. It’s easy to spot these when the result is mediocre overall, at least to someone who is used to coaching and teaching; it becomes much harder when it feels brilliant, but part of it is completely made-up. Then it is like reading Theranos founder Elizabeth Holmes; you’re unsure whether what is said is true or not.
Therefore, it should always be a human saying the final word on anything created by AI for the foreseeable future. This obviously prevents us from self-driving cars for a while (either the autopilot has the final say or the car isn’t self-driving), but it also puts the blame on AI-written texts and images to whoever published it.
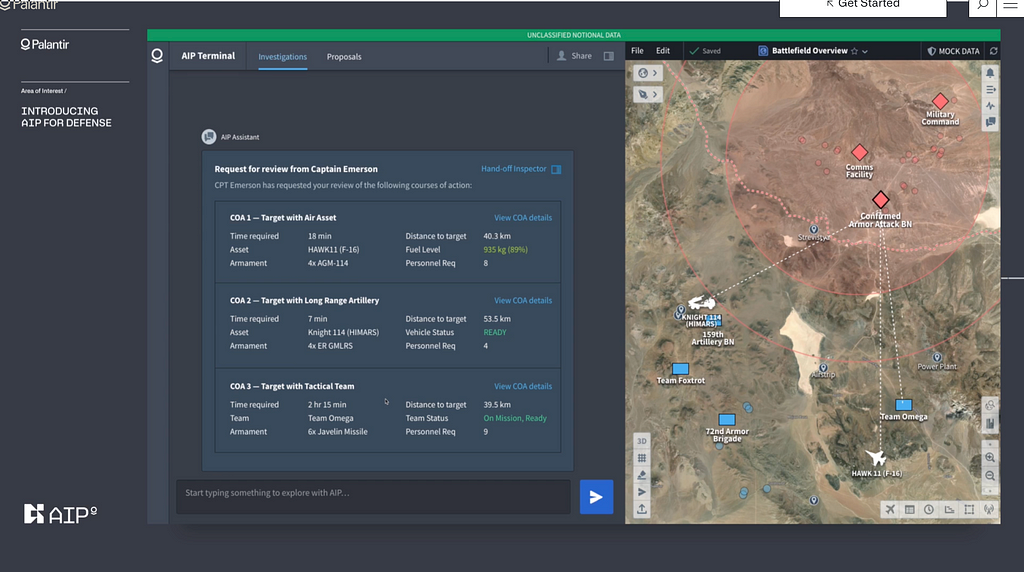
Prototyping is mainly through prompt engineering, and we are awaiting the tools which learn from local context, eg. Google AI Studio and Tailwind is a good place to start.
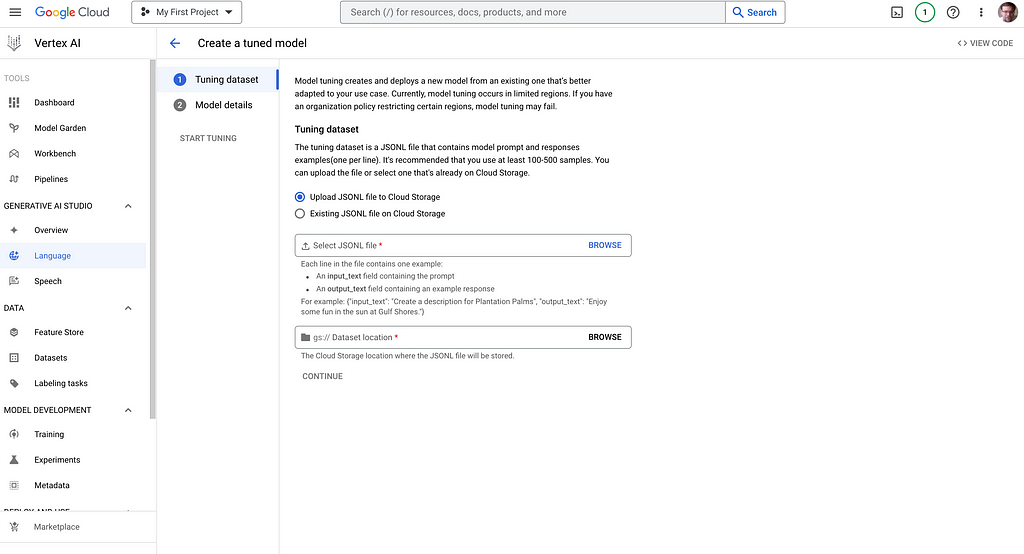
AI-enabling products with AI cases
It’s easy: check your journeys!
Whether it’s business processes, service blueprints, user or customer journeys or standard operation procedures, you should always check what activities you could outsource it to a different (robotic) colleague.
Always look for the largest chunks first
- Can the whole job (to be done..) be automated?
- Can a role someone does in it be completely automated?
- Can some activities be automated?
- Can certain tasks be solved through AI?
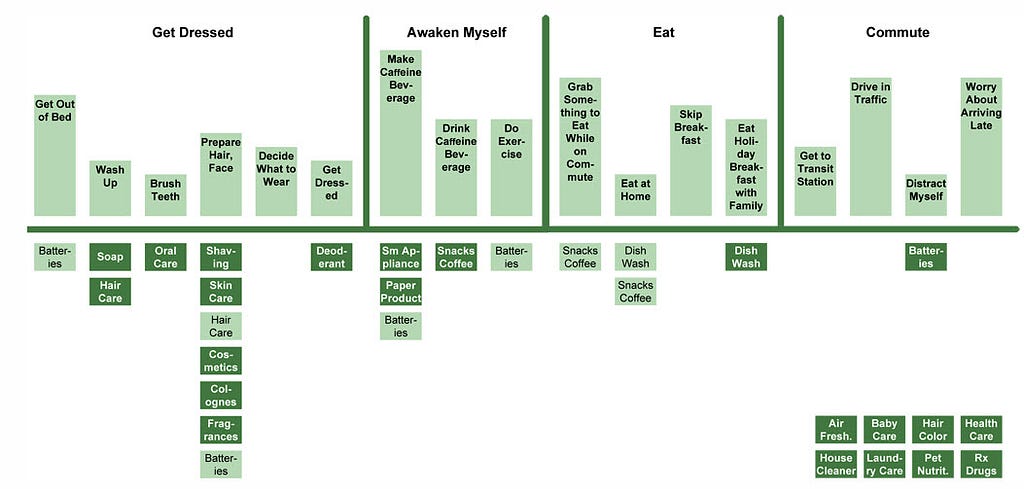
You should go for the bigger chunk first. It’s great if you have a car able to detect edges while parking, better yet if it parks itself, but obviously, one which drives itself trumps all of these features. I would rather buy a car which drives itself from home to destination parking lot but can’t park, than one which can park, but can’t drive. Obviously the best is when both are provided and I can just use it as a taxi, replacing drivers completely.
Prompt through Slack
It’s very easy to prototype certain tasks using the Wizard of Oz method: ask someone to do it over Slack or Messenger! Check:
- what contextual information or preliminary training was needed
- what were the edge cases
- if there was any error or misunderstanding, how was it corrected
- what kind of tools were used
If you have a good enough set of prompts and answers, you might be able to literally feed an AI with it.
Write clever prompts to ChatGPT / Bard / Bing
And lastly, you can always use ChatGPT as a prototyping playground. Helps a lot of tasks and it even helps you think! At least if you buy the plus version…
The three cases of AI enablement in UX was originally published in UX Collective on Medium, where people are continuing the conversation by highlighting and responding to this story.
Leave a Reply