Successful AI products enable both to do their best work, Framing problems for AI, from mechanisms to capabilities
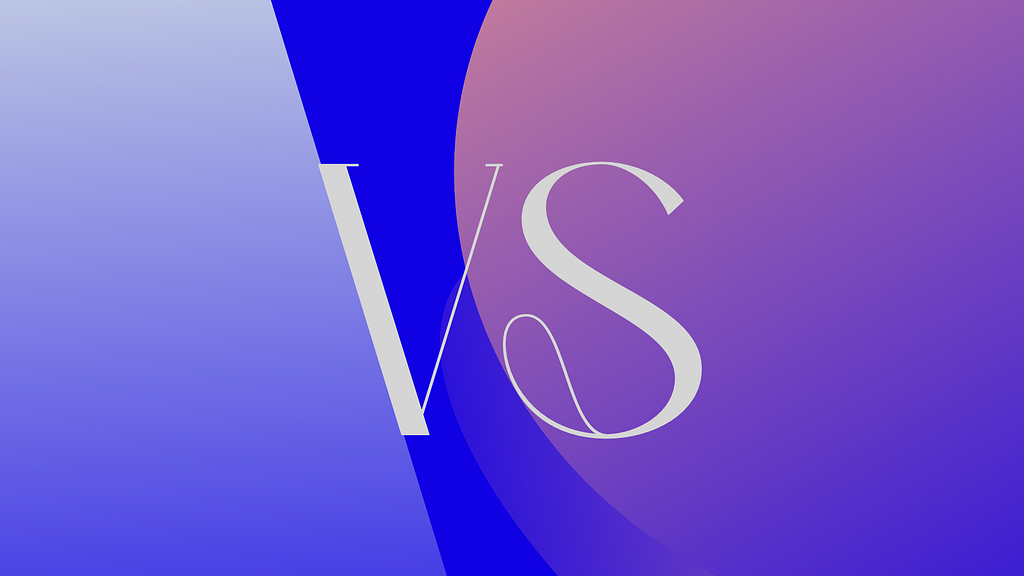
There are tasks humans can do, tasks AI can automate, and a new category of tasks humans can do only with the help of AI, source: Turing Trap.
Successful AI products enable both to do their best work. Points below frame ways to approach building AI capabilities toward useful, valuable, responsibly considered human needs
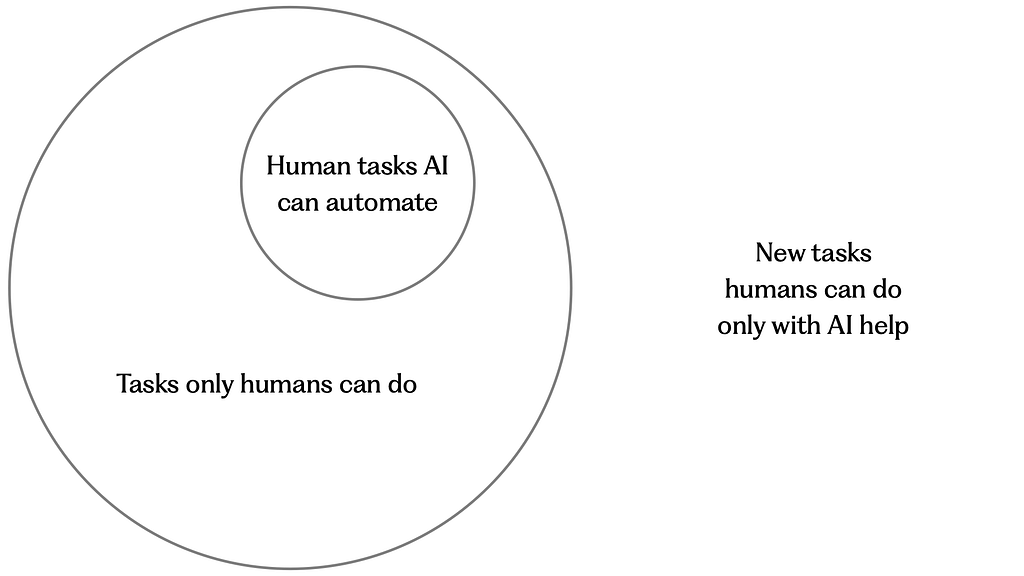
Data Science and Design have grown from different disciplines, but converge when productizing data-driven capabilities, when AI is called to attention in interfaces.
Building great AI products starts from identifying valuable opportunities. However, literature surrounding AI has traditionally focused on mechanisms, how AI works: GANs, Neural Nets, RAG, etc. There’s little on capabilities, what AI can do for human tasks other than replacing them.
Referred to as the AI Innovation gap, Data Science and ML experts tend to be far removed from users to think of ideas people want, building capabilities looking for a problem to solve. Designers approach problems from the user’s perspective, but have limited understanding of AI’s qualities, coming up with ideas that can’t be built.
AI products need both. From Sam Stone’s Unlearning to Build Great AI Apps, simultaneously work backwards from user problems, and forwards from technology opportunities.
Successful AI products match technology capability with the right human problem to solve.
Any of three points below position humans and AI to each do their best work. This framing simultaneously begins to avoid concerns for fairness, ethics, unintended consequences because they present opportunities where each uniquely excels, instead of human substitutions:
(1) AI does something in a different way from what exists today,
(2) AI does something new that couldn’t have be possible before,
(3) AI is better than nothing, accomplishing things people don’t want to do or care for doing or have had to do in the past
1. AI does something in a different way
Before smart watches, people manually logged physical activity. Now you can record physical activity anytime, anywhere. Before mobile, people called taxis from phone books. Now a ride is one tap away.
Re-imagine the same tasks
Within existing categories, AI can do the same things differently. For example, instead of browsing through 20 results mostly related with what you’re looking for, teleport directly to the exact result with AI. Specify the outcome and skip to the end with intent-based outcome specification, first introduced in 1993.
Another example: instead of taking notes during a meeting, have a transcription run in the background and mark moments in time. Just like taking photos to capture a moment, come back to revisit key moments later. Outcome is generally the same, the way to do it gets a lot easier, and more fun.
Explain & evaluate benefits
When coming up with AI capabilities that do the same task differently, evaluate benefits and worst case scenarios. Human capability substitutions, giving credit to artists for generated content, freedom of choice for recommender systems… are tricky from a responsible AI perspective, when ultimately in the hands of users.
They say to explain AI when designing for users. But remember people ultimately care about the benefit, not the technology, unless knowing is crucial to understand & use the product. From a former colleague, “opening the camera app, I don’t care how lighting exposure mechanisms work when I press the button. Just let me preserve a memory.”
Dependent on use case, explaining what’s under the hood is more important in some situations (fraud detection, cancer prediction) than others (music recommendations, object detection from images).
There’s less need to explain when everything goes right. More need to explain when (1) things go wrong (2) root cause needed for tracing decisions, (3) moderating user expectations given AI’s capability uncertainty.
2. AI does something new, not possible before
Consider AI products that leverage AI’s uniqueness: accuracy, speed, scale… Examples of precedent widely range from real-time language translation to drug discovery.
Difficult tasks, Unsafe tasks, Impossible tasks
When coming up with things AI can do & valuable for people, start from tasks people cannot do, or are unsafe for people to do.
Difficult tasks involve things like sifting through big data in seconds, detecting patterns & insights that would take weeks to recognize if at all
Unsafe tasks involve things like inspecting a transmission tower & high-radiation electrical assets, detecting anomalies of aging infrastructure, ultimately preventing power outages for a city, see 3D tower capture
Impossible tasks involve retaining long term memory across a person’s lifetime, remembering and recalling your notes from the day you took them, see mem.ai
Handoff Moments
Sometimes a collaborative partnership may be better suited than either AI or humans alone. For example, AI can analyze medical images and patient data, then leave it up doctors for decisions. AI can navigate vehicles in standard road environments, handing off to the driver when anticipating unexpected situations or weather conditions. AI can take a first pass flagging inappropriate content on social media, handing off to people for review.
Research believes AI is still decades away from human sentience. Industry hypes it’s coming soon.
Right now, AI can do an OK job, not an expert job. At the same time, AI has unique qualities people don’t have, enabling new tasks we’re coming up with.
3. AI is better than nothing
When AI excels where traditional technology solutions fall short: A well-researched & validated area are tasks that are repetitive, mundane, or undesirable for humans, a.k.a. unremarkable AI
AI’s incredible capabilities feel increasingly human: It can clone your voice, see your screen (ReALM), detect autism, make music videos, assist driving, fold laundry… usually followed by concerns about appropriateness, questioning ‘what makes us human’
Rather than replicating another human task as the starting point for data exploration, consider situations where unremarkable AI can offer outsized results & convenience
Unremarkable AI & computing
These fit well toward enterprise use cases. They are the experiential improvements, low-hanging fruit people tend to miss because they are designed to go unnoticed, temporary, fitting in the user’s flow of actions naturally, appearing only in key moments. To remark would be to notice something unusual, mismatching expectations.
Inspired from unremarkable computing, & Ambient Intelligence concepts applied to home IoT systems, they intend to make technology invisible & unobtrusive, augmenting routines so people can focus on what’s important.
Automating the tedious, redundant, manual
Similarly, unremarkable AI can offload (1) tedious tasks prone to human error, (2) redundant, manual tasks with speed, at scale
Tedious tasks are highly routine, mentally or physically taxing over time, have minimal change & intellectual stimulation. These are administrative actions like scheduling appointments, customer support answering common FAQs, counting historical sales data and warehouse inventory, legal document reviews..
Redundant tasks are repeatable things people do: filling forms, opening music playlist at the gym, editing photos and videos, catching up on emails. Simple AI solutions that can automate them, such as landing people on the most likely tab, smart defaults, autofill. See 6 adaptive patterns, using common UI components.
Considerations
History suggests with every emergent technology, people tend to assign human traits. It’s harder to come up with non-human AI capabilities where AI is uniquely valuable, often leading to building working AI systems still in search for the right problem match to be made & become productized.
Successful AI products tend to fall into situations where AI does (1) the same thing differently, (2) something new not possible before, (3) or better than nothing.
These are categories where AI is best positioned to succeed, leveraging AI’s unique qualities, not necessarily replacing human tasks but re-imaging tasks best suited for each role.
Thanks for reading! I’d love to know your thoughts.
Elaine writes about design, AI, emergent tech. Follow for more, connect on LinkedIn.
Human vs. Machine: framing the right problems for AI to solve was originally published in UX Collective on Medium, where people are continuing the conversation by highlighting and responding to this story.
Leave a Reply